One of the privileges of being on the campus of the Massachusetts Institute of Technology (MIT) in Cambridge is seeing glimpses of the future, from advances in quantum computing and energy sustainability and production, to designing new antibiotics. Do I understand it all deeply? No, but I am able to wrap my head around much of it when I am asked to create an image to document the research.
The joy of being a science photographer is that I must learn about the things I am documenting to produce communicative and trustworthy images, intended as a form of data, for the researchers who welcome me into their laboratories.
But now, with the wide availability of generative artificial intelligence (genAI) tools, lots of questions must be asked. Will there be a point at which, with just a few keystrokes and prompts, a scientist can create a ‘visual’ of their research, as I do with my camera, and consider that image a record of the work? Will researchers, journals and readers be able to spot artificially created images and understand that they do not truly document the work? And finally, from a personal point of view, will there still be a place for a science photographer like me to advance the communication of research? Here’s what I have found out while experimenting with artificial intelligence (AI) image generators.
Reality and representation
First, let’s remind ourselves of the differences between a photograph, in which each pixel corresponds to real-world photons, and a genAI visual, created with a diffusion model — a complex computational process that generates something that seems real but might never have existed.
To explore these differences, I decided to experiment with genAI visuals made with Midjourney and OpenAI’s DALL-E diffusion models to reproduce the work shown in one of my most popular science photographs — with help from scientific-visualization researcher Gaël McGill at Harvard University in Cambridge, Massachusetts.
In 1997, Moungi Bawendi, a chemist at MIT, asked me to take a picture of his nanocrystals (quantum dots). When excited with ultraviolet light, these crystals fluoresce at different wavelengths depending on their size. Bawendi, who later shared a Nobel prize for this work, did not like the first image (see ‘Three views’), in which I had placed the vials flat on the lab bench, taking a top-down photograph. You can tell that was how I had placed them, because you can see the air bubbles in the tubes. It was intentional; I thought it made the image more interesting.
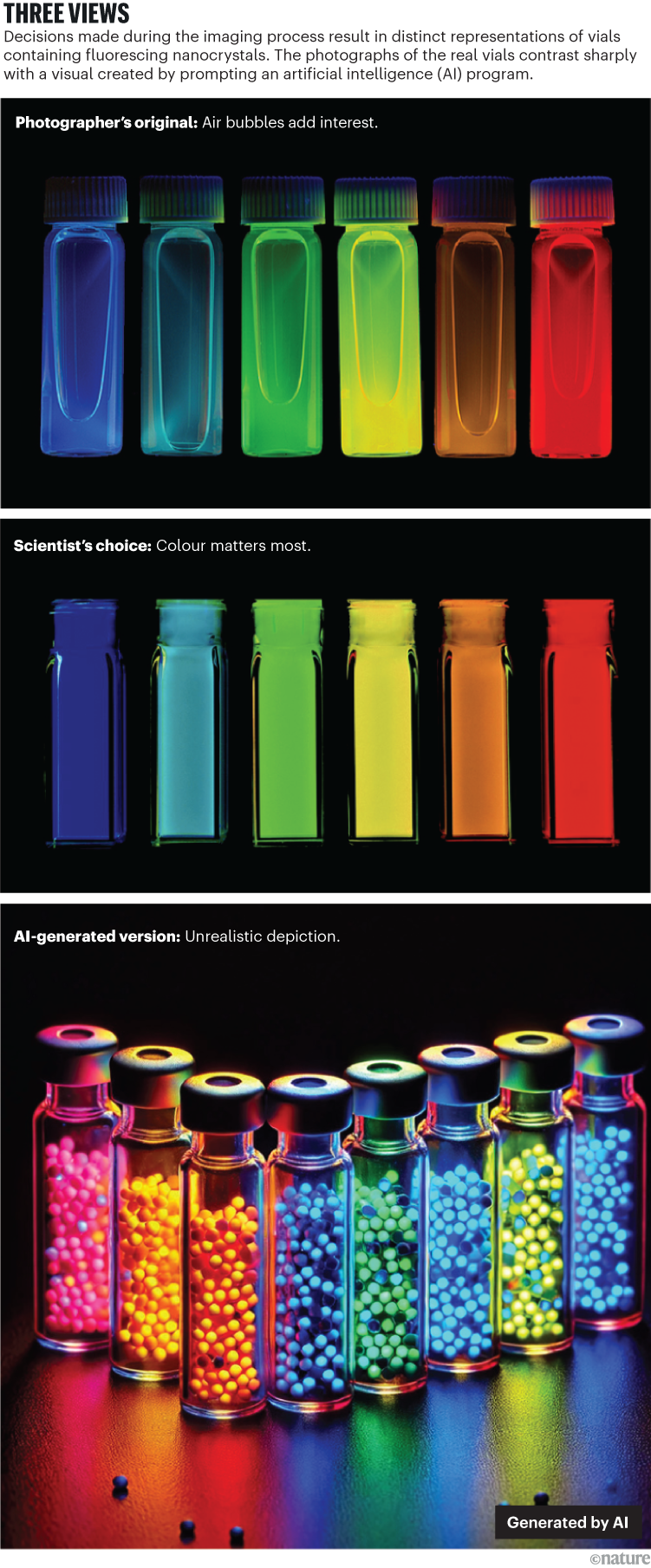
Credit: Felice Frankel
The second iteration was used on the November 1997 cover of the Journal of Physical Chemistry B (see ‘Three views’). That photograph provides a straightforward record of the research and highlights the importance of collaborating with the scientist — an essential part of my process.
To generate a comparable image in DALL-E, I used the prompt “create a photo of Moungi Bawendi’s nanocrystals in vials against a black background, fluorescing at different wavelengths, depending on their size, when excited with UV light”.
China made waves with Deepseek, but its real ambition is AI-driven industrial innovation
People might think that the image the program produced is attractive (see ‘Three views’), but it is not even close to the reality captured in the original photograph. DALL-E introduced bead-like dots that were not in the prompt. The algorithm presumably found the words ‘quantum dots’ in the data set of the AI model that underlies it and used that information to replace the words “nanocrystals”.
More troubling is the fact that, in each vial, there are dots with different colours, implying that the samples contain a mix of materials that fluoresce at a range of wavelengths — this is inaccurate. Furthermore, some of the dots are shown lying on the surface of the table. Was that an aesthetic decision made by the model? I find the resulting visual fascinating (see Supplementary Information).
The results of my AI experimentation are often cartoon-like images that can hardly pass as reality — let alone documentation — but there will be a time when they will be. In conversations with colleagues in research and computer-science communities, they all agree that we should have clear standards on what is and is not allowed. In my opinion, a genAI visual should never be allowed as documentation.
Manipulated versus AI generated
The advent of AI means that we need to clarify three core issues related to visual communication: the difference between illustration and documentation, the ethics of image manipulation and the desperate need for visual-communication training for scientists and engineers.
Decisions about how to frame an image — what to include or leave out — are already a manipulation of reality. The tools that people decide to use are also part of the manipulation. Each digital camera creates a distinct photograph. An Apple iPhone’s algorithm enhances image colours differently to that of a Samsung phone. Similarly, the near-infrared images produced by the James Webb Space Telescope are designed to be different from, yet complement, the Hubble Space Telescope’s optical scans.
How AI is unlocking ancient texts — and could rewrite history
Taking the point even further, the colours we see in all of those amazing images of the Universe are digitally enhanced and give us yet more renditions of reality. Seen through this lens, it is clear that humans have been, in effect, artificially generating images for years, without necessarily labelling them as such. However, there is a crucial difference between enhancing a photograph with software to depict reality and creating a reality from trained data sets.
As a science photographer, I am acutely aware of the difference between an illustration and a documentary photograph, but I am less confident that AI programs can make this distinction. An illustration or diagram is a representation of something, subjectively translating and visually describing a concept or structure using notations, colours, shapes and so on. A documentary optical photograph, or one made using scanning or transmission electron microscopy, is created with photons and electrons and is therefore a representation of an item, even if it is not the item itself. The difference between the two is in the intent.
With illustration, the intent is to describe and clarify the work. GenAI visuals will probably excel in that task. But for a documentary photograph, the intent is to bring us as close to reality as we can. Both are, in essence, already a form of manipulation or an act of artificial generation, and therein lies the importance of defining and discussing their ethics before we include genAI tools.
Publishers now have software in place to identify various manipulations in images that already exist (see Nature 626, 697–698; 2024), but, frankly, AI programs will eventually be able to circumvent these fail-safes. There are efforts under way to find ways to trace the provenance of a photograph or to document any manipulation of the original. For example, the forensic photography community, through the global Coalition for Content Provenance and Authenticity, provides technical information to camera manufacturers regarding the ability to trace the provenance of a photograph by keeping a record in the camera of any manipulation. As one can imagine, not all manufactures are on board.
Are the Internet and AI affecting our memory? What the science says
The scientific community still has time to create a system of transparency and form guidelines regarding AI-generated images. At a minimum, every genAI visual should be clearly labelled as such, and the process and tools used to create it should be clearly stated and include, where possible, credit for any source images provided to the AI engine. However, listing the sources poses a challenge.
Two articles have raised an important issue by highlighting potential privacy and copyright violations when using diffusion models (N. Carlini et al. Preprint at arXiv https://doi.org/grqmsb (2023); and see go.nature.com/4jqyevn). Credit is only feasible in a closed system (which diffusion models are not) for which the training data are known and fully documented. For example, Springer Nature, which publishes Nature (Nature is independent of its publisher), has recently included an exception into its policy for Google DeepMind’s AlphaFold program to cover this sort of use (for models trained on a specific set of scientific data). However, people should keep in mind that AlphaFold is not a genAI tool that creates images — it generates structural models (coordinate data) that are then turned into images by people (not by genAI tools).
Happily, efforts are addressing privacy issues. Creators can now use a kind of ‘tamper-evident’ metadata called Content Credentials to, as Adobe explains in its manual, “obtain proper recognition and promote transparency in the content creation process” (see go.nature.com/3wx92ng).
Ethical standards
For years, I have suggested that scientists need to be trained in the ethics of visual communication, and the easy availability of AI image-creation software adds urgency to this discussion.
For example, I recall one experience with an engineer who altered a photograph that I had made of their research and wanted to publish it, along with the submitted article (see Supplementary Information). The researcher did not consider that altering the image was, in fact, similar to changing their data because they had not been taught the basic ethics of image manipulation and visual communication.