The smell in the laboratory was new. It was, in the language of the business, tenacious: for more than a week, the odour clung to the paper on which it had been blotted.
To researcher Alex Wiltschko, it was the smell of summertime in Texas: watermelon, but more precisely, the boundary where the red flesh transitions into white rind.
“It was a molecule that nobody had ever seen before,” says Wiltschko, who runs a company called Osmo, based in Cambridge, Massachusetts. His team created the compound, called 533, as part of its mission to understand and digitize smell. His goal — to develop a system that can detect, predict or create odours — is a tall order, as molecule 533 shows. “If you looked at the structure, you would never have guessed that it smelled this way.”
That’s one of the problems with understanding smell: the chemical structure of a molecule tells you almost nothing about its odour. Two chemicals with very similar structures can smell wildly different; and two wildly different chemical structures can produce an almost identical odour. And most smells — coffee, Camembert, ripe tomatoes — are mixtures of many tens or hundreds of aroma molecules, intensifying the challenge of understanding how chemistry gives rise to olfactory experience.
Another problem is working out how smells relate to each other. With vision, the spectrum is a simple colour palette: red, green, blue and all their swirling intermediates. Sounds have a frequency and a volume, but for smell there are no obvious parameters. Where does an odour identifiable as ‘frost’ sit in relation to ‘sauna’? It’s a real challenge to make predictions about smell, says Joel Mainland, a neuroscientist at the Monell Chemical Senses Center, an independent research institute in Philadelphia, Pennsylvania.
How do we smell? First 3D structure of human odour receptor offers clues
Animals, including humans, have evolved a remarkably complex decoding system befitting the enormous repertoire of odour molecules. All sensory information is processed by receptors, and odour is no different — except in its scale. For light, the human eye has two types of receptor cell; for smell, there are 400. How the signals from these receptors combine to trigger a particular perception is unclear. Plus, the receptor proteins themselves are hard to work with, so what they look like and how they function has mostly been guesswork.
Things are beginning to change, however, thanks to improvements in structural biology, data analytics and artificial intelligence (AI). Many scientists hope that cracking the olfactory code will help them to understand how animals use this essential sense to find food or mates, and how it feeds into memory, emotion, stress, appetite and more.
Others are trying to digitize smell to build new technologies: devices that diagnose disease on the basis of odours; better, safer insect repellents; and affordable or more-effective aroma molecules for the US$30-billion flavour and fragrance market. At least 20 start-up firms are trying to make electronic noses for applications in health and public safety.
This all adds up to a surge of research into the biology of olfaction, says Sandeep Robert Datta, a neuroscientist at Harvard Medical School in Boston, Massachusetts. “Smell is having a moment,” he says.
Smelling machines
Even for experts, the physical properties of an odour molecule typically offer little insight into how it will actually smell.
Researchers have come up with a few computational models that can relate structure to odour, but early versions tended to be based on quite narrow data sets or could only make predictions when smells had been calibrated to have the same perceived intensity. In 2020, one team reported a model that could predict how similar real-world mixtures were to each other, correctly identifying that rose and violet odorants are more similar to one another than either is to the pungent spice asafoetida, often used in Indian cuisine1.
Previous attempts to use machine learning were good, but not great. For example, when researchers ran a competition to create the best odour-predicting model, algorithms from 22 teams could effectively predict only 8 out of 19 smell descriptors2.
Could rats and dogs detect disease better than the finest lab equipment?
Last year, Wiltschko’s team — then part of Google’s AI research division — collaborated with researchers at Monell, including Mainland, to publish a map for smell3 that made use of AI.
Their program was trained by feeding the model thousands of descriptions of molecular structures from fragrance catalogues, along with smell labels for each — terms such as ‘beefy’ or ‘floral’.
Then, the researchers compared the AI system with human noses. They trained 15 panellists to rate a few hundred aromas using 55 labels, such as ‘smoky’, ‘tropical’ and ‘waxy’.
Humans have a hard time with this task because smell is so subjective. “There’s no universal truth,” says Mainland. Most smell descriptions lack detail, too. For one smell, panellists chose the words ‘sharp, sweet, roasted, buttery’. A master perfumer, asked to describe the same smell, noted ‘ski lodge, fireplace without a fire’. “That shows you the gap,” says Mainland. “Our lexicon is not good enough.” Nonetheless, a human panel is one of the best available tools for coming up with consistent smell descriptors because the average rankings of the group for different smells tend to be stable.
Using the structure of these molecules alone, the AI algorithm did well at predicting the smell of compounds compared with the average group assessments (see ‘Same but different’), and it performed better than the typical individual sniffer. And although the map it produced was very complicated — it has more than 250 dimensions — it was able to group smells by type, such as meaty, alcoholic or woody.
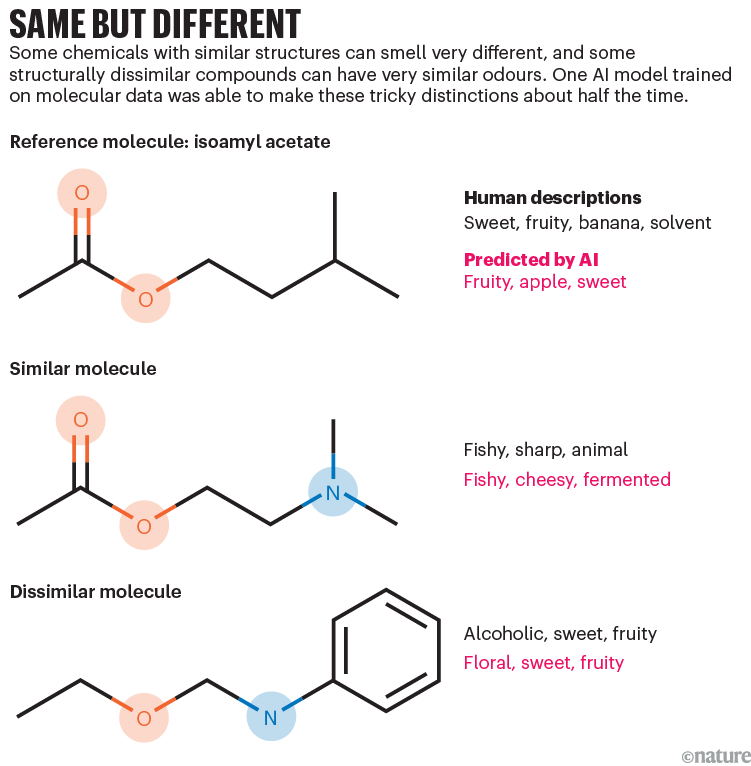
Source: Ref. 3
Mainland says that the algorithm’s thoroughness helped it to perform. Humans might rate an odour as fruity but forget to rate it as sweet. The model, exhaustive and patient, churns through all possibilities each time.
One challenge that both Mainland and the Osmo team are working on now is to work out whether the model can predict what mixtures of compounds smell like, on the basis of their components. Another goal is to have the model design new odours, for instance chemicals that mimic a specific scent, or that are safer, more sustainable or biodegradable.
AI probably can’t do this alone, says Jane Parker, a flavour chemist at the University of Reading, UK, who helped the smell-mapping team with quality control of their compounds. “The model could give you an idea of what might work,” she says. But the expertise and ingenuity of human chemists and flavourists — plus their highly trained noses — will still be necessary for innovation.
Mysterious code
For both expert and amateur sniffers, the biological equipment for smell detection is the same. The nose has millions of olfactory neurons, and each typically expresses just one type of odorant receptor (OR). The gene family that encodes them was discovered4 in the early 1990s and won Linda Buck and Richard Axel a Nobel prize in 2004.
Each of these receptor types might recognize one or more odorants — and each odorant might be recognized by more than one receptor. Together, the roughly 400 human ORs can respond to a trillion different chemicals. It’s a fiendishly complex, exquisitely tuned, flexible system — and it needs to be, because the chemistry of nature is incredibly diverse, says Aashish Manglik, a biochemist at the University of California, San Francisco. “The breadth of chemicals that make odours is enormous.” One important step in cracking the code of smell is to know what the receptors look like and how they recognize chemicals. But they have been notoriously difficult to study. “They’re the most recalcitrant membrane proteins to work with,” says Manglik. Many are too unstable to be expressed in cells in the lab and to generate enough protein to analyse.
Scientists have deciphered the structure of two ORs from insects5,6. These receptors are of a totally different type to those in mammals, although the olfactory ‘logic’ by which they work together is likely to be similar, says sensory neuroscientist Vanessa Ruta, whose lab at the Rockefeller University in New York solved both the structures.
Two more receptor structures7,8, from the olfactory system in mice, followed last year. Both of them sense a bunch of chemicals with distinctly unlikeable fishy, musky or putrid odours, many of which are key components of bodily odours in animals.
Getting at these structures has required some “funky approaches”, says Manglik, because ORs are so hard to grow in the lab. But last year, he was part of a team that succeeded in publishing the first protein structure of a human olfactory receptor bound to an odorant9.
Having tried almost every OR that they could, Manglik and colleagues found one that is richly expressed outside the nose, in the gut and prostate, and which could, as a result, be made more readily in commonly used cell lines. It’s a receptor called OR51E2 and it responds to the chemical propionate, which has a pungent, cheesy odour.
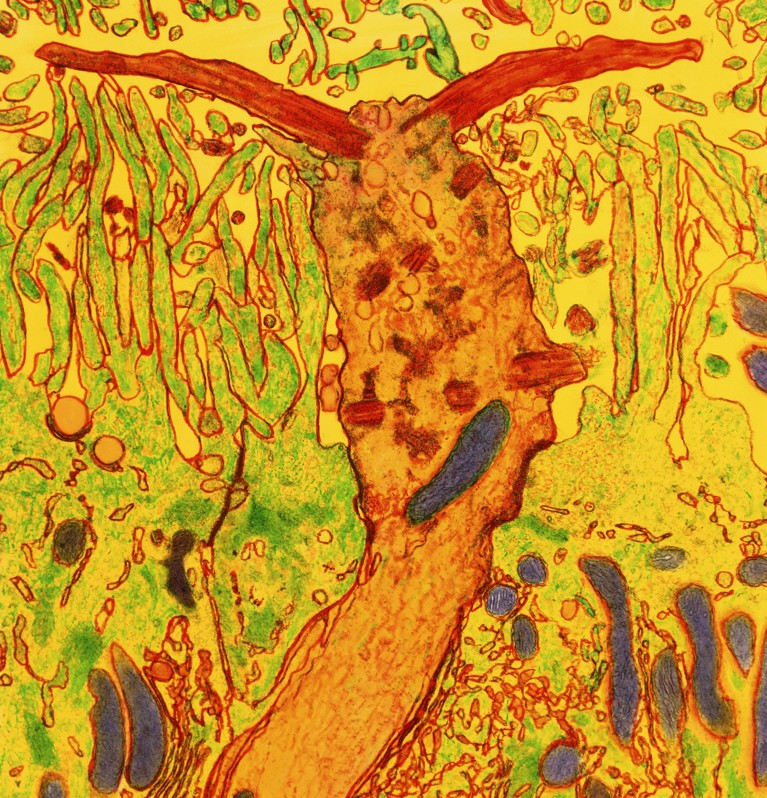
The olfactory receptor cell (orange) is a neuron with cilia (red) that reach into the nasal cavity.Credit: Prof. P. Motta/Dept. of Anatomy/University “La Sapienza”, Rome/SPL
Using cryo-electron microscopy, the team looked at how propionate binds to the receptor in a little pocket, and how that binding changes the receptor’s shape and conveys information onwards. Seeing the structure “was really thrilling”, says Buck, whose lab at the Fred Hutchinson Cancer Center in Seattle, Washington, studies olfactory neuroscience.
But ORs can detect so many odorants that “the structure of one OR can’t tell us much”, says Hiro Matsunami, an olfaction biologist at Duke University in Durham, North Carolina, who collaborated with Manglik on the study of OR51E2.
Alongside trying to grow more of them, Matsunami and his colleagues have attempted to understand the OR by re-engineering it. They made some synthetic receptors using OR51E2 and parts from two dozen similar receptors. They aligned the amino-acid sequences of these existing ORs, and chose the most frequent amino acid at each position to build an average, or ‘consensus’, structure. Then they expressed the structure in cells. When they compared their synthetic structure with its real-life counterpart, OR51E2, it looked and behaved just like its sibling10.
Next, they tried building another averaged receptor based on an OR with no published structure — OR1A1 — which recognizes a broad range of odorants including some that smell fruity, floral and minty. They used a computational model to explore how it bound to two compounds that both smell of menthol; the compounds bound to the receptor in different places.
The team thinks that different odorants probably engage a single OR in distinct ways. That would help to explain the level of complexity in the smell code — and could explain why, for example, two disparate chemicals can have similar odours, or why chemically similar compounds can smell so different. The compound carvone, for instance, comes in two varieties that are mirror images of each other; one smells of spearmint, the other of caraway or dill. “There must be a receptor that can explain this,” says Matsunami.
Some researchers are using machine learning to accelerate the search for structures and their preferred chemical partners. Right now, scientists have identified odour molecules that bind to only about 20% of human ORs.
The protein-prediction algorithm AlphaFold has suggested thousands of structures for mammalian odorant receptors11. And machine learning and modelling has helped Matsunami and his colleagues to screen millions of compounds to see which ones might bind to two candidate OR structures12. One of the molecules they found smells of orange blossom; another strongly of honey.
The dream end point is to gather data on hundreds of ORs and how their activation lines up with the chemistry of millions of odorants, says Manglik.
Lead with the nose
Once a smell is processed by receptors, this information proceeds to a brain region called the olfactory bulb, which sits behind the bridge of the nose, and onwards to the olfactory cortex. The circuitry for olfaction before information enters the cortex is well understood, particularly in model organisms such as fruit flies and mice. But the olfactory cortex is more of a mystery. “It’s difficult to figure out what’s happening there,” says Buck.
Many researchers want to understand how information from receptors is organized in the brain, and what rules govern perception. If that were understood, it might be possible to get an animal to perceive a certain smell without even presenting an odorant, simply by recreating the pattern it generates in the brain, says Dima Rinberg, a neuroscientist who studies smell at New York University School of Medicine.
Human nose can detect 1 trillion odours
Another big unknown, Datta says, is how the olfactory system interacts with other crucial brain circuits, such as those that control movement or navigation. Several labs, including his own, are interested in how animals actively sense scent and move towards or away from odours.
Capturing the connection between scent and behaviour is already possible to some extent in the brains of insects. In fruit flies, for instance, scientists can explore chemical structure, receptors and the brain in a single system. “In insects you can start to span the whole spectrum,” says Ruta.
Insects’ sense of smell is also relevant to human health. Mosquitoes evolved to sniff out humans, and many insects prey on crops that humans rely on. Last November, Osmo announced a $3.5-million grant from the Bill & Melinda Gates Foundation in Seattle, Washington, aimed at discovering and producing compounds that repel, attract or destroy insects that carry disease.
Meanwhile, detecting scents is also big business. For some tasks and applications, ‘electronic noses’ are already commercially available: some are designed to detect off odours in food or to pick up smells in waste water. They are being intensively studied as diagnostics for diseases such as TB, diabetes and various cancers.
But natural sniffers still have an edge, and even without a full understanding of how brains process smell, scientists can exploit biological noses to improve chemical sensing for safety, security or health care.
The classic example is the sniffer dog, widely used to sense chemicals in explosives or narcotics — but these animals are expensive to train and there are limits to what they can detect.
Rinberg’s team is aiming to blend animal and digital odour detection. They developed a nose–computer interface in mice13, using electrodes that record signals from the olfactory bulb as mice smell different compounds. The researchers can decode odour identities from neural activity, and then use the patterns to flag these smells under natural conditions. Their device, which is now being developed by a start-up called Canaery, co-founded by Rinberg, retains the precision of the animal’s sense of smell without researchers having to train the animal to respond. “The biological nose is the best chemical detector,” Rinberg says. “The whole machinery is hard to beat.”
Despite the pre-eminence of biology, many scientists dream of a time when a digital smell sensor will rival those for other senses. “Smartphones can do image and audio recognition,” says Ruta. “But for olfaction there’s nothing like that.”
And although they know how well biological noses work, researchers still have a lot of outstanding questions. For Buck, the simplest to pose could be the most difficult to answer. “It would be nice to know how you get a perception of a particular odorant,” she says — how the brain, beyond the nose, creates a sense of rose, for instance, and how it distinguishes it from the essence of fish. “How does that happen in the brain? No one knows,” she says. “We don’t have the techniques to figure that out yet.”