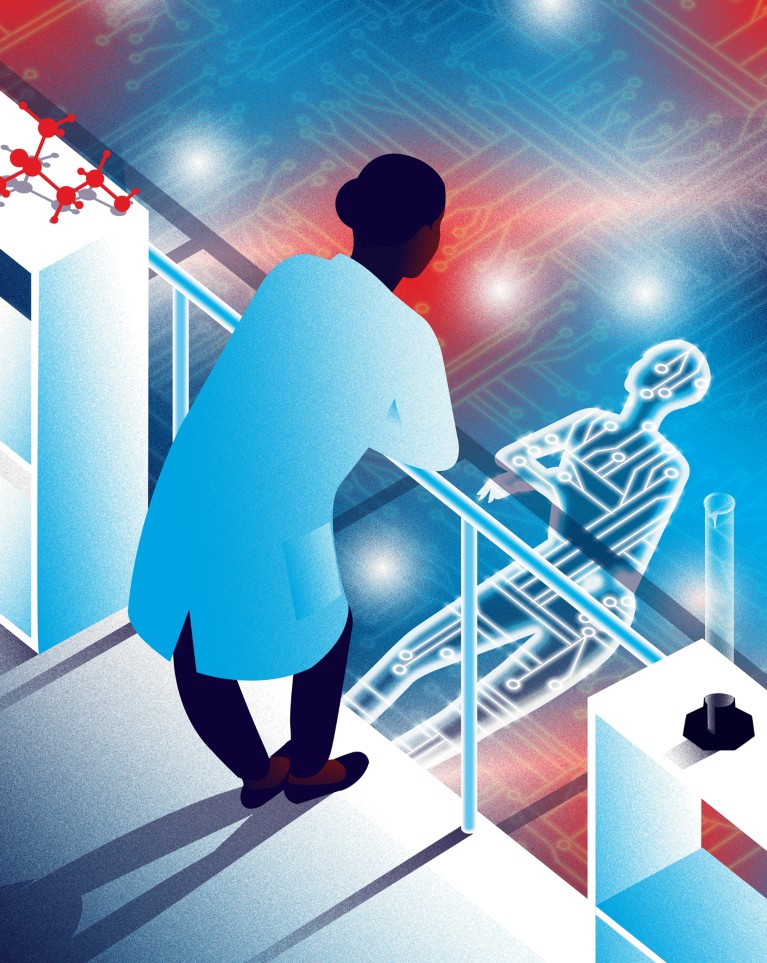
Illustration: Neil Webb
The current boom in artificial intelligence (AI) would probably not exist were it not for work that began in academia. Many of the techniques that are now being used on an everyday basis, such as machine learning and natural-language processing, are underpinned by academic work into artificial neural networks that dates back decades. But it is true to say that much of the latest cutting-edge and high-profile research in AI is being done not in university labs, but behind the closed doors of private companies.
“We’re increasingly looking at a situation where top-notch AI research is done primarily within the research labs of a rather small number of mostly US-based companies,” says Holger Hoos, an AI researcher at RWTH Aachen University in Germany.
Nature Index 2024 Artificial intelligence
Much of this work is not published in leading peer-reviewed scientific journals. In 2023, research by corporations accounted for only 3.84% of the United States’ total Nature Index output in AI. But data from other sources show the increasingly influential role that companies play in research. In a paper published in Science1 last year, Nur Ahmed, who studies innovation and AI at the Massachusetts Institute of Technology in Cambridge, and his colleagues, found that research articles with one or more industry co-author grew from 22% of the presentations at leading AI conferences in 2000 to 38% in 2020. Industry’s share of the biggest, and therefore most capable, AI models went from 11% in 2010 to 96% in 2021. And on a set of 20 benchmarks used to evaluate the performance of AI models — such as their capabilities in image recognition, sentiment analysis and machine translation — industry alone, or in collaboration with universities, had the leading model 62% of the time before 2017, a share that has grown to 91% since 2020. “Industry is increasingly dominating the field,” says Ahmed.
That growing dominance of the outputs of AI research is largely a result of industry’s massive advantage in funding. In 2021, US government agencies (excluding the Department of Defense) spent US$1.5 billion on AI research and development, and the European Commission spent €1 billion (US$1.1 billion). Industry worldwide spent more than US$340 billion.
This outlay has given industry a stranglehold on the three most important inputs: computing power, large data sets and talent, says Ahmed.
Companies have access to much greater computing power than academic institutions, including the ability to buy the graphics-processing units (the most common chips used in AI) they need, or even design and manufacture their own. This allows firms to create much larger and more complex models than their academic counterparts. In 2021, industry AI models were 29 times bigger, on average, than academic models.
Companies also have access to much larger data sets with which to train those models because their commercial platforms naturally produce that data as users interact with them. “When it comes to training state-of-the-art large language models for natural-language processing, academia is going to be hard-pressed to keep up,” says Fabian Theis, a computational biologist at Helmholtz Munich, in Germany.
Lucrative salaries, and the promise of being able to work on the cutting edge of AI technology allows companies to snap up much of the top talent from universities, while hiring inside academic computer-science departments has remained largely flat.
“Industry hiring is much higher than the overall growth of computer science research faculty,” says Ahmed. In 2004, just 21% of AI PhDs at North American universities went to work in industry, but by 2020, that number was almost 70%. This growing imbalance worries some in academia. The biggest concern is that companies are by necessity focused on profits, which influences not only the kinds of AI products they seek to develop, but also the research questions they ask in the first place. “If developments of major consequence for society are driven primarily by short-term commercial interests, we have a problem,” says Hoos.
Academic AI research is needed to contribute to the development of a body of knowledge that did not originate from a commercial imperative, says Shannon Vallor, who studies the ethics of AI at the University of Edinburgh, UK. “Academia is the only place where researchers still have the ability to work without an obvious roadmap to profit,” she says.
Academics can provide a critical and dispassionate view on AI and be an independent source of information on what works and what doesn’t, as well as identifying the potential harms of new technologies and how to mitigate them, says Vallor. Academics can also help to align AI research with what is in the public interest. “At the moment, there is a deficit of AI applications focused on the kinds of problems we most need to address,” says Vallor — including challenges such as climate change, health-care needs, and the social and democratic stresses that have been amplified by digital technologies.
Despite the importance of engaging with the ethical and social consequences of AI, many scholars are concerned that, because of the incentive structures in place in industry, firms are underinvesting in research into the responsible use of AI and failing to incorporate the lessons from such research. An analysis2 by Ahmed and other colleagues confirms that suspicion. Leading AI firms have significantly lower output for responsible-AI research compared with conventional AI papers. The responsible-AI research they do perform is also narrower in scope and lacks diversity in the topics addressed.
“Major AI companies demonstrate minimal public engagement in responsible-AI research, indicating that speed is prioritized over safety in AI development,” says Ahmed. They also found a disconnect between responsible-AI research and its practical implementation. “The AI products reaching the market show limited influence from responsible-AI research findings,” says Ahmed.
Companies had invested more heavily in responsible-AI research in the past, says Vallor, but that interest waned with the boom in generative AI, prompting a “race to the bottom” to capitalize on the market. “The knowledge about responsible AI is all there, the problem is that large AI companies don’t have incentives to apply it,” she says. “But we could change the incentives.”
Companies that develop and deploy AI responsibly could face a lighter tax burden, she suggests. “Those that don’t want to adopt responsible-AI standards should pay to compensate the public who they endanger and whose livelihoods are being sacrificed,” says Vallor.
As we wait for new regulations, academia has an important role to play in keeping an eye on its industry colleagues. Academic studies that identify and offer solutions for issues such as the inherent biases built into AI systems are needed to help the field develop in a more responsible direction. “There need to be checks and balances and they cannot be achieved by regulation alone, there also needs to be scrutiny by independent experts,” says Hoos. “It’s crucial that similar expertise to that of industry exists at publicly funded institutions, like universities.”
For that scrutiny to happen, however, it is imperative that academics have open access to the technology and code that underpins commercial AI models. “Nobody, not even the best experts, can just look at a complex neural network and figure out exactly how it works,” says Hoos. “We know very little about the capabilities and limitations of these systems, so it is absolutely essential that we know as much as possible about how they are created.”
Theis says many companies are making moves towards open access for their AI models, because they want more people to be able to work with them. “It’s a core interest for industry to have people trained on their tools,” he says. Meta, the parent company of Facebook, for example, has been pushing for more open models because it wants to better compete with the likes of OpenAI and Google. Giving people access to its models will allow an inflow of new, creative ideas, says Daniel Acuña, a computer scientist at the University of Colorado Boulder.
But it is unrealistic to expect that companies will give away all of their “secret sauce”, says Hoos — another reason it is important that academia retains the capability, in both technology and talent, to keep up with industry developments.
Mutual benefits
Not everyone is overly concerned with industry dominating parts of AI development, as they expect academics and companies to find their way to an equilibrium. “It needs to be clear that there are benefits for both sides” of industry and academia being heavily involved in AI research, says Theis.
Companies benefit from the freedom that academics have to pursue unexpected or high-risk research directions, which could result in novel breakthroughs that solve some of the problems their products face. “Some of the limits of the current AI tools may not be overcome without a radically different approach,” says Vallor. And that approach is more likely to be found by researchers who are less concerned with whether their ideas can be turned into a successful product.
Academics, for their part, although they are free to pursue curiosity-driven projects, can also gain knowledge and support from industry to help them solve interesting and tricky problems. “It’s very common for trainees from my and other labs to go to big tech, or pharma, to learn about the industry experience,” says Theis. “There’s actually a back and forth and diffusion between the two.”
Acuña and his colleagues have studied the different approaches of industry and academic researchers to AI3. They analysed papers presented at a variety of AI conferences between 1995 and 2020 to see how the composition of a research team was related to the novelty of their work, and its impact in terms of citations and models created.
They found that work by teams comprising solely of industry researchers tends to be more highly cited and to result in state-of-the-art models. Academic teams, in contrast, tend to produce higher novelty work, with their papers more likely to contain unconventional and atypical ideas. Interestingly, academic–industry collaborations tend to see similar results to industry teams, working on difficult engineering problems that attract lots of citations but losing the novelty that is the hallmark of academic projects.
This division of labour, familiar to many other fields of science, is why Acuña says he is more optimistic than some others about the future of AI research in academia. Even if academics don’t have the resources or computing power to build the biggest large language models, they have the ability to do work that is even more novel and ground-breaking. “Just go crazy,” he says. “Don’t disregard a field just because you’re in academia, you have the freedom to do whatever you want.”
Keeping pace with industry
To make the most of that freedom, however, academics will need support — most importantly in the form of funding. “A strong investment into basic research more broadly, so it is not just happening in a few eclectic places, would be useful,” says Theis.
Although governments are unlikely to be able to match the huge amounts of money being splashed around by industry, smaller, more focused investments can have outsized influence. “Canada’s AI strategy hasn’t cost a ton of money, but has been very effective,” says Hoos. The country has invested around Can$2 billion (US$1.46 billion) in AI initiatives since 2016, and in 2024 announced plans to spend another Can$2.4 billion over the next few years. Much of that money is earmarked for providing university researchers with access to the computing power they need for AI applications, to support responsible AI research and to recruit and retain top talent. This strategy has contributed to Canada’s ability to punch above its weight and remain near the top of the global leaderboard in both academic research and commercial development. It placed 7th in the world for Nature Index output in AI research in 2023, and 9th in natural sciences overall.
Recruitment programmes such as the Canada Excellence Research Chairs initiative, which offers up to Can$8 million over eight years to entice top researchers in various fields to move to, or remain in, Canada, and Germany’s Alexander von Humboldt Professorships in AI, worth €5 million over five years, have both helped to shore up AI research in the countries. Hoos himself holds one of the Humboldt professorships.
Europe is also home to several initiatives to boost academic research in AI. Theis is scientific director of Helmholtz AI, an initiative run by the Helmholtz Association of German Research Centres. The unit provides funding, computing access and consulting for research labs to help them apply AI tools to their work, such as finding new ways of using the large data sets they produce in areas such as drug discovery and climate modelling. “We want to enable researchers in AI by democratizing access to it,” says Theis. “To really accelerate those research labs.”
An even more ambitious plan has been put forward by CLAIRE, the Confederation of Laboratories for Artificial Intelligence Research in Europe, which was co-founded by Hoos in 2018. The plan is inspired by the approach in physical sciences of sharing large, expensive facilities across institutions and even countries. “Our friends the particle physicists have the right idea,” says Hoos. “They build big machines funded by public money.”
Hoos and his colleagues in CLAIRE have proposed a ‘moonshot’ plan to create a facility to provide the computing infrastructure necessary for academic scientists to keep pace with industry when it comes to AI research — a sort of CERN for AI, referring to the particle-physics laboratory near Geneva, Switzerland. They estimate that the project would require around €100 billion in funding from the European Union over six years, an amount Hoos says is quite reasonable compared with the cost of the original moonshot — NASA’s Apollo space programme, which cost about €240 billion in today’s money — and to CERN itself. Such a facility would be used to do AI research ‘out in the open’, rather than in private company labs, he says, making it fully transparent to the public. And just like the Apollo programme and CERN, it would have great benefits to both society and industry, he says.
Whatever approach is taken, keeping publicly funded, independent academic researchers at the forefront of AI progress is crucial for the safe development of the technology, says Vallor. “AI is a technology that has the potential to be very dangerous if it’s misused, if it doesn’t have the right guardrails and governance, and if it’s not developed in responsible ways,” she says. “We should be concerned about any AI ecosystem where the commercial incentives are the only ones driving the bus.”