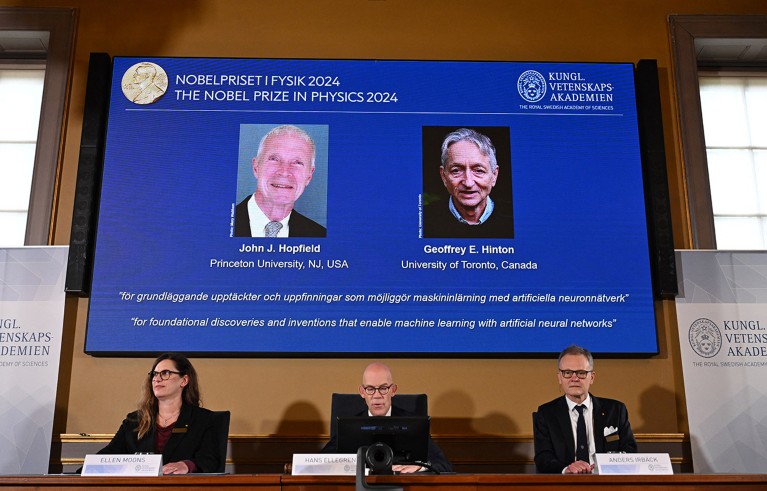
The winners were announced by the Royal Swedish Academy of Sciences in Stockholm.Credit: Jonathan Nackstrand/AFP via Getty
Two researchers who developed machine-learning techniques that underpin today’s boom in artificial intelligence (AI) have won the 2024 Nobel Prize in Physics.
John Hopfield from Princeton University in New Jersey, and Geoffrey Hinton at the University of Toronto, Canada, share the 11 million Swedish kroner (US$1 million) prize, announced by the Royal Swedish Academy of Sciences in Stockholm on 8 October.
Both used tools from physics to come up with methods that power artificial neural networks, which use brain-inspired, layered structures to learn abstract concepts. Their discoveries “form the building blocks of machine learning, that can aid humans in making faster and more reliable decisions”, said Nobel committee chair Ellen Moons, a physicist at Karlstad University, Sweden, during the announcement. “Artificial neural networks have been used to advance research across physics topics as diverse as particle physics, material science and astrophysics.”
Computer science: The learning machines
In 1982, Hopfield, a theoretical biologist with a background in physics, came up with a network that described connections between nodes as physical forces1. By storing patterns as a low-energy state of the network, the system could recreate the image when prompted with a similar pattern. It became known as associate memory, because of its similarity to the brain trying to remember a rarely-used word or concept.
Hinton, a computer scientist, later used principles from statistical physics, which is used to collectively describe systems made up of too many parts to track individually, to further develop the ‘Hopfield network’. By building probabilities into a layered version of the network, he created a tool that could recognise and classify images, or generate new examples of the type it was trained on2.
These processes differed from computation that came before it, as the networks were able to learn from examples, including from unstructured data, that would have been challenging for conventional software based on step-by-step calculations.
The networks are “grossly idealized models that are as different from real biological neural networks as apples are from planets”, Hinton wrote in Nature in 2000. But they proved useful and have been built upon widely. Neural networks that mimic human learning form the basis of many state-of-the-art AI tools, from large language models (LLMs) to machine-learning algorithms capable of analyse large swathes of data, including the protein-structure-prediction model AlphaFold.
Speaking by telephone at the announcement, Hinton said that learning he had won the Nobel was “a bolt from the blue”. “I’m flabbergasted, I had no idea this would happen,” he said. He added that advances in machine learning “will have a huge influence, it will be comparable with the industrial revolution. But instead of exceeding people in physical strength, it’s going to exceed people in intellectual ability”.
This is a breaking news story that will be updated throughout the day.
Additional reporting by Helena Kudiabor.