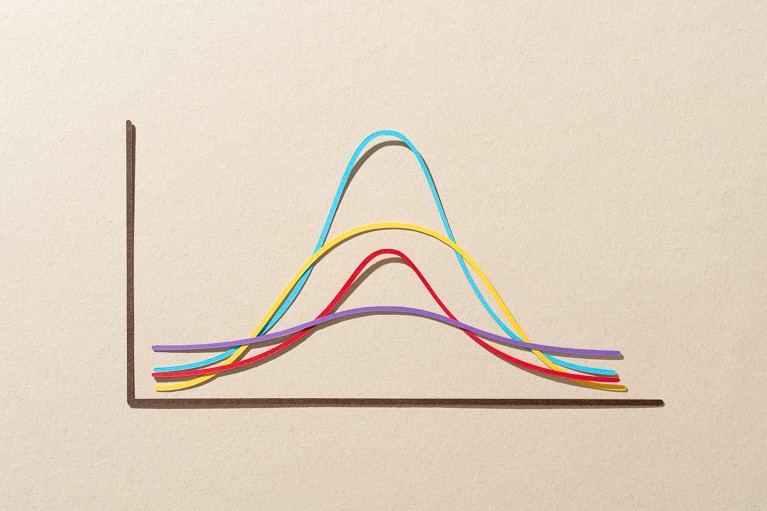
Credit: MirageC / Getty
It can happen so easily. You’re excited about an experiment, so you sneak an early peek at the data to see if the P value — a measure of statistical significance — has dipped below the threshold of 0.05. Or maybe you’ve tried analysing your results in several different ways, hoping one will give you that significant finding. These temptations are common, especially in the cut-throat world of publish-or-perish academia. But giving in to them can lead to what scientists call P hacking.
P hacking is the practice of tweaking the analysis or data to get a statistically significant result. In other words, you’re fishing for a desirable outcome and reporting only the catches, while ignoring all the times you came up empty. It might get you a publication in the short term, but P hacking contributes to the reproducibility and replicability crisis in science by filling the literature with dubious or unfounded conclusions.
Most researchers don’t set out to cheat, but they could unknowingly make choices that push them towards a significant result. Here are five ways P hacking can slip into your research.
Ending the experiment too early
You might plan to gather 30 samples but find yourself running a quick analysis halfway through, just to see where things stand. If you notice a statistically significant difference after 15 samples, you might be inclined to stop the experiment early — after all, you’ve found what you were looking for.
It’s time to talk about ditching statistical significance
But stopping an experiment once you find a significant effect but before you reach your predetermined sample size is classic P hacking. It’s like declaring the winner of an election after polling just half the electorate: the result might not be representative of reality. What’s the solution? Decide on the sample size or data-collection process ahead of time and stick to it, no matter how eager you are to see the results.
Running experiments until you get a hit
Another often-unintentional form of P hacking is repeating the experiment or analysis until you obtain a statistically significant result. Imagine you run an experiment and the outcome is insignificant. You try again with a new batch of samples — still nothing. You repeat the study once more, and voila! P < 0.05. Success? Not quite. If you selectively report only the attempt that ‘worked’ and ignore those that didn’t, you’re engaging in P hacking by omission. As any gambler knows, if you roll the dice often enough, eventually you’ll get the result you want by chance alone (not that I’m a gambler). The better approach is to report all the experimental replicates, including those that didn’t work.
Cherry-picking your results
A less benign form of P hacking is selective reporting. Imagine you measure several outcomes or observe your effect at multiple time points — for instance, testing a therapy’s impact on recipients’ blood pressure, cholesterol, weight and blood sugar regularly over an entire month. After analysing the data, you find that only one outcome — say, blood sugar at week 3 — showed a significant improvement. You might be tempted to highlight this one promising result and downplay the rest, or even omit them from your report. This is cherry-picking: by showing only the favourable data and ignoring everything else, you create a biased narrative.
In this example, people might think the therapy worked because it lowered blood sugar at week 3, even though the overall data are not so rosy. Putting these data into the paper’s supplementary material and continuing with the experiment on the basis of this one finding is also a no-no. You should report all relevant results, not just the ones that support the hypothesis. Science progresses faster when we know what doesn’t work, as well as what does.
Tweaking your data
In data analysis, you often have to make judgements about what to include, what to exclude and how to report the data. P hacking can sneak in when those decisions are guided by the desire to achieve significance rather than by scientific reasoning. For example, you might notice an outlier in your data set. Including it in the analysis gives you a P value of 0.08, whereas excluding it brings P down to 0.03. Problem solved? Not quite.
In these cases, it is best practice to go back to the original data or laboratory notes to determine whether experimental conditions could explain this outlier. Perhaps you pipetted double the amount of reagent into your sample, or construction work nearby during the time you were testing that animal affected its behaviour. Researchers can often rationalize their data-filtration decisions, and most of those decisions are warranted. But if the real motive is to turn an insignificant result into a significant one, it crosses into questionable territory. The key is to decide on data-filtering rules before looking at the results. If, for some reason, you have to make a change after data collection, explain that — and say why.