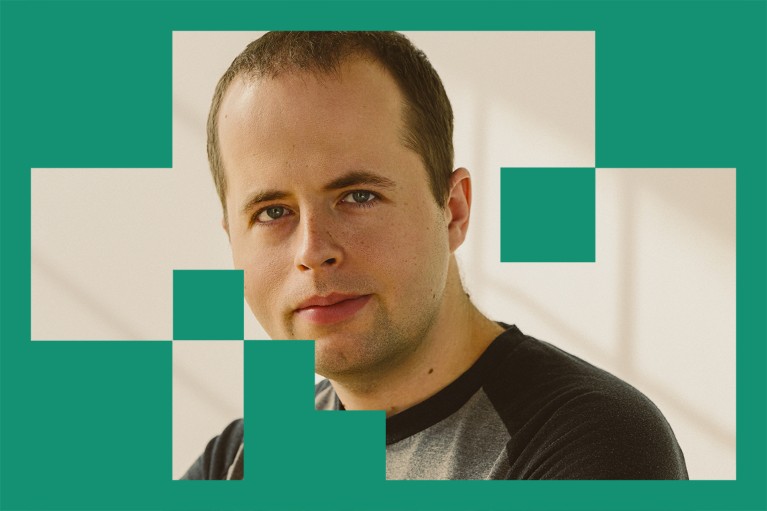
Jakub Pachocki has been OpenAI’s chief scientist since 2024.Credit: OpenAI
OpenAI is best known for ChatGPT — the free-to-use, large language model-based chatbot that became a household name after its debut in 2022. The firm, in San Francisco, California, has since released a string of cutting-edge artificial intelligence (AI) tools, including ‘reasoning’ models that use step-by-step ‘thought’ processes to specialize in logical tasks.
OpenAI’s ‘deep research’ tool: is it useful for scientists?
These tools have helped researchers to polish prose, write code, review the literature and even generate hypotheses. But, like other technology rivals, OpenAI has faced criticisms over the energy demands of its models and the way in which data are exploited to train their models. And unlike some firms, OpenAI has almost exclusively released proprietary models that researchers can use, but can’t build on.
Jakub Pachocki has been the firm’s chief scientist since 2024. He joined OpenAI in 2017 from academia, where he was a theoretical computer scientist and competitive programmer. Now, he leads the development of the company’s most advanced AI systems, which are designed to tackle complex tasks in science, mathematics and coding.
Nature spoke to Pachocki about whether AI can generate original science, artificial general intelligence (AGI) and the firm’s upcoming open-weight model.
Scientists are increasingly using reasoning models. What role could these models have in five years?
Today you can talk to a model, but it’s only an assistant that needs constant guidance. I expect this will be the primary thing that changes.
We already see that something like OpenAI’s Deep Research [a tool that can synthesize reams of information] is capable of working unsupervised for 10 or 20 minutes and producing something useful. But the amount of compute that’s going into solving these queries is tiny.
What are the best AI tools for research? Nature’s guide
If you have open research problems it is worth spending much more compute on that. I expect we will have AIs that actually are capable of novel research. We’ll see a lot of progress, for example, on autonomous software engineering and the autonomous design of hardware components, and similar applications in other disciplines.
How important has reinforcement learning — the iterative learning process based on trial, error and reward — been in creating OpenAI’s reasoning models?
The original ChatGPT releases involved an unsupervised pre-training stage, where the model just ingests a massive amount of data and builds a sort of ‘world model’. Out of that, we extract a useful assistant through a process of reinforcement learning, using human feedback.
One way you can view this latest progress on reasoning models is that itreally increases the emphasis on the reinforcement-learning phase, so that we’re not only extracting something but also enabling the model to find its own way of thinking.
How much energy will AI really consume? The good, the bad and the unknown
A question is whether we should continue to think of these stages of learning separately. Reasoning models don’t learn how to think in a vacuum, they are rooted in a model that has learned through pre-training. A lot of my focus is on thinking about that phase and combining the approaches and understanding their interaction.
You talk about the models ‘thinking’. Do models actually reason? Or do they approximate something that looks like reasoning?
One thing that we should be clear about is that the way the models work is different from how a human brain works. A pre-trained model has learned some things about the world, but it doesn’t really have any conception of how it learned them, or any temporal order as to when it learned things.