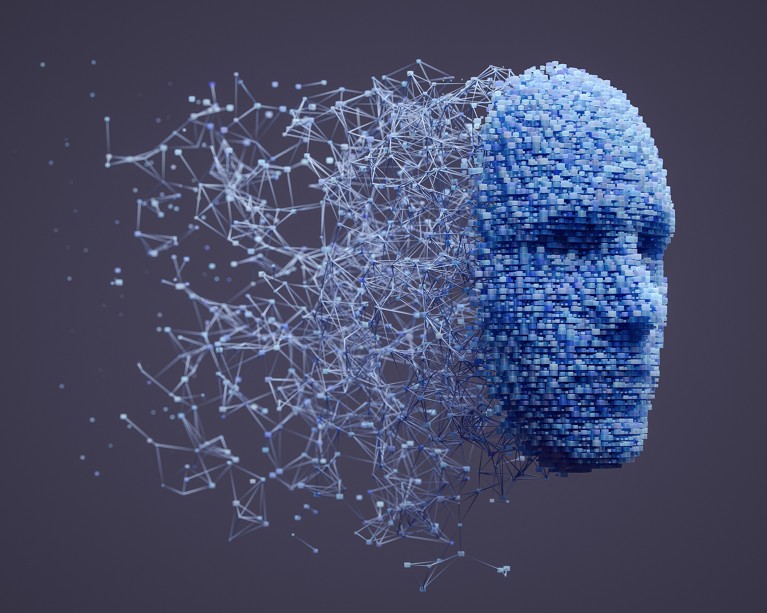
Some researchers think AI systems will reach human-level intelligence soon; others think it’s far away.Credit: Getty
The technology firm OpenAI made headlines last month when its latest experimental chatbot model, o3, achieved a high score on a test that marks progress towards artificial general intelligence (AGI). OpenAI’s o3 scored 87.5%, trouncing the previous best score for an artificial intelligence (AI) system of 55.5%.
How close is AI to human-level intelligence?
This is “a genuine breakthrough”, says AI researcher François Chollet, who created the test, called Abstraction and Reasoning Corpus for Artificial General Intelligence (ARC-AGI)1, in 2019 while working at Google, based in Mountain View, California. A high score on the test doesn’t mean that AGI — broadly defined as a computing system that can reason, plan and learn skills as well as humans can — has been achieved, Chollet says, but o3 is “absolutely” capable of reasoning and “has quite substantial generalization power”.
Researchers are bowled over by o3’s performance across a variety of tests, or benchmarks, including the extremely difficult FrontierMath test, announced in November by the virtual research institute Epoch AI. “It’s extremely impressive,” says David Rein, an AI-benchmarking researcher at the Model Evaluation & Threat Research group, which is based in Berkeley, California.
ChatGPT broke the Turing test — the race is on for new ways to assess AI
But many, including Rein, caution that it’s hard to tell whether the ARC-AGI test really measures AI’s capacity to reason and generalize. “There have been a lot of benchmarks that purport to measure something fundamental for intelligence, and it turns out they didn’t,” Rein says. The hunt continues, he says, for ever-better tests.
OpenAI, based in San Francisco, has not revealed how o3 works, but the system arrived on the scene soon after the firm’s o1 model, which uses ‘chain of thought’ logic to solve problems by talking itself through a series of reasoning steps. Some specialists think that o3 might be producing a series of different chains of thought to help whittle down the best answer from a range of options.
Spending more time refining an answer at test time makes a huge difference to the results, says Chollet, who is now based in Seattle, Washington. But o3 comes at a massive expense: to tackle each task in the ARC-AGI test, its high-scoring mode took an average of 14 minutes and probably cost thousands of dollars. (Computing costs are estimated, Chollet says, on the basis of how much OpenAI charges customers per token or word, which depends on factors including electricity usage and hardware costs.) This “raises sustainability concerns”, says Xiang Yue at Carnegie Mellon University in Pittsburgh, Pennsylvania, who studies large language models (LLMs) that power chatbots.
Generally smart
Although the term AGI is often used to describe a computing system that meets or surpasses human cognitive abilities across a broad range of tasks, no technical definition for it exists. As a result, there is no consensus on when AI tools might achieve AGI. Some say the moment has already arrived; others say it is still far away.
Many tests are being developed to track progress towards AGI. Some, including Rein’s 2023 Google-Proof Q&A2, are intended to assess an AI system’s performance on PhD-level science problems. OpenAI’s 2024 MLE-bench pits an AI system against 75 challenges hosted on Kaggle, an online data-science competition platform. The challenges include real-world problems such as translating ancient scrolls and developing vaccines3.
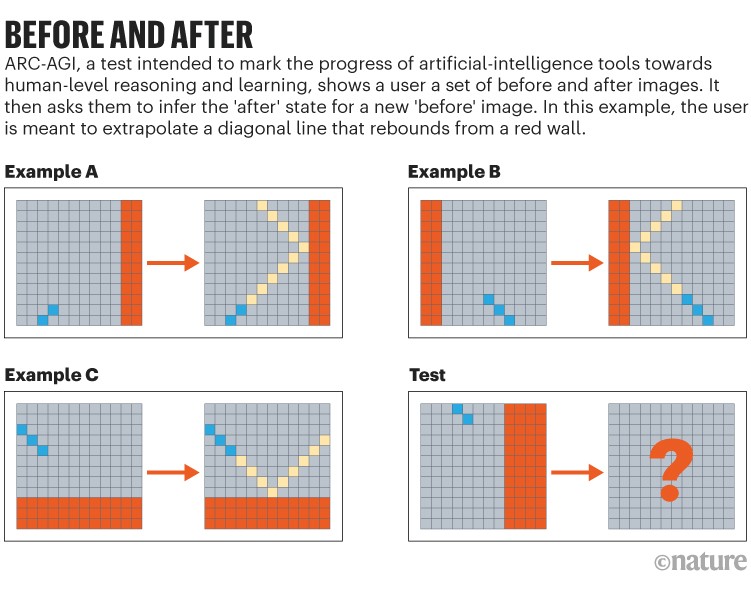
Source: Ref. 1
Good benchmarks need to sidestep a host of issues. For instance, it is essential that the AI hasn’t seen the same questions while being trained, and the questions should be designed in such a way that the AI can’t cheat by taking shortcuts. “LLMs are adept at leveraging subtle textual hints to derive answers without engaging in true reasoning,” Yue says. The tests should ideally be as messy and noisy as real-world conditions while also setting targets for energy efficiency, he adds.
Yue led the development of a test called the Massive Multi-discipline Multimodal Understanding and Reasoning Benchmark for Expert AGI (MMMU), which asks chatbots to do university-level, visual-based tasks such as interpreting sheet music, graphs and circuit diagrams4. Yue says that OpenAI’s o1 holds the current MMMU record of 78.2% (o3’s score is unknown), compared with a top-tier human performance of 88.6%.
The ARC-AGI, by contrast, relies on basic skills in mathematics and pattern recognition that humans typically develop in early childhood. It provides test-takers with a demonstration set of before and after designs, and asks them to infer the ‘after’ state for a novel ‘before’ design (see ‘Before and after’). “I like the ARC-AGI test for its complementary perspective,” Yue says.