For clinicians on the challenging front line of chronic pain treatment, the tale of a general-practice physician in Australia who sought specialist help for her chronic migraines will be all too familiar.
Her ferocious migraines would strike with daily frequency. “She would vomit and have to lie down between patient consults,” says Marc Russo, a pain-medicine physician at Hunter Pain Specialists in Broadmeadow, Australia, who treated her in consultation with a neurologist. “Then she’d have to get up, rinse her mouth and see the next patient.”
After coming under Russo’s care, it took three long years to find a way to control her migraines. The problem was not a shortage of options — there are plenty of treatments for people with chronic migraines, Russo says. However, finding the right treatment for each person with chronic pain is currently an excruciating game of trial and error, testing treatments one by one in the hope of finding a match.
Nature Outlook: Pain
“You have to give each drug at least six weeks to see if it works, before trying the next one,” Russo says. Each drug can cause side effects, whether or not it eases the pain. After cycling through each migraine drug individually, the team started testing drug combinations. “We finally got control of her migraines on the 24th medication trial,” Russo says.
Today, there are dozens of non-opioid drugs with pain-relieving (analgesic) benefits, as well as a variety of non-pharmaceutical options for chronic pain relief, such as electronic spinal-cord implants, physiotherapy and cognitive behavioural therapy. But the guesswork means that chronic pain care is still a highly frustrating process for patients and clinicians, says Sean Mackey, a physician–researcher in pain medicine at Stanford University in California. “The issue is not that we’re short of quality pain treatments,” Mackey says. “The issue is that we don’t know how to match them up to the right patient.”
There is growing evidence, however, that biomarkers that can be detected in brain scans and blood tests could be predictive of treatment efficacy. This could enable physicians to more precisely tailor pain treatments to individuals — a practice that has already revolutionized cancer therapy. “Personalized pain medicine is no longer science fiction,” Mackey says. “We can now see a real way forward to help people.”
Agonizing complexity
Objectively measuring a person’s pain is more challenging than tracking a more tangible ailment, such as the growth of a tumour. Pain is not only subjective, but also by its nature, highly complex.
“Pain is a biopsychosocial condition,” says Jan Vollert, a chronic-pain researcher studying pain biomarkers at the University of Exeter, UK. “Both in its generation and appreciation, there are factors that go beyond what we can measure in your body or blood.” In addition to the initial physical trigger of pain, factors such as a person’s social network, job status, past trauma and resilience can all strongly affect how pain is experienced, how debilitating it is and how long it lasts. “With cancer, whether or not you have a malignant growth is a yes or no answer,” says Vollert. “Pain is much more complicated.”
For Karen Davis, a neuroscientist at the Krembil Brain Institute at Toronto Western Hospital in Canada, the psychosocial aspect of chronic pain became clear in the late 1980s. She was working as a postdoc at the Johns Hopkins Blaustein Pain Treatment Center in Baltimore, Maryland, and saw that many people’s chronic pain had started with an injury such as a fractured bone. Most people would soon recover from this and the pain would cease. “It was very clear that the same initiating factors led to different outcomes in different people,” says Davis.
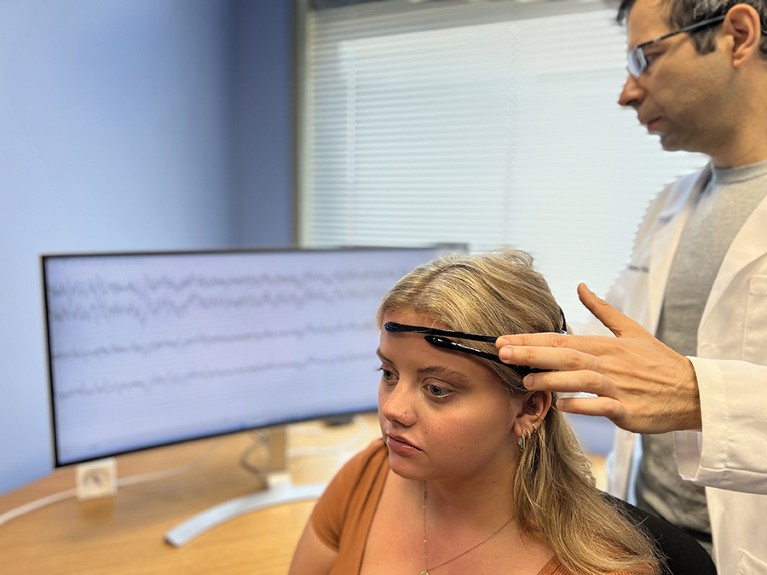
Algorithms can make predictions about pain state from electroencephalogram signals.Credit: Saab Lab. (formerly STAR), Cleveland Clinic
An optimistic person who breaks their arm mountain biking on a weekend away with friends to celebrate a promotion could experience pain differently to a pessimistic person who breaks an arm cycling from their monotonous job to their home, on which they are behind on the mortgage repayments. Anxiety and depression can be risk factors for the onset of chronic pain. “Nobody experiencing chronic pain is in the same place at the same time,” she says. “That pain is situated in a milieu of everything else going around them, everything they’ve previously experienced, everything they’re anticipating, and all the emotions involved in that experience.”
These human complexities make efforts to capture pain in the brain difficult, let alone being able to identify biomarkers that would guide physicians to the best treatment for each person. In animals, pain biomarkers can be found in the brain by using an electroencephalogram (EEG)1, says Carl Saab, founder and scientific director at the Cleveland Clinic Consortium for Pain in Ohio. “We get a very clear signature of pain in mice, rats, dogs and non-human primates, and this signal is mostly preserved, consistent and stable,” he says. In humans, however, EEG readings from people experiencing pain are all over the place. “Pain is one of the most heterogeneous clinical conditions I know,” says Saab. “Your pain is different than mine, because your approach to life is different — you might worry about your family more than me, or your job — and so when I average you with 50 other people, that signal is going to get washed out.”
Washed out to the human eye, at least. “When we compared the EEGs of people with pain versus those with no pain, standard statistical analysis showed no difference,” Saab says. When Saab and his collaborators trialled a basic form of machine learning to process the data, however, the artificial intelligence (AI) algorithm could detect something. The algorithm could differentiate not only between EEGs from people with chronic lower back pain and healthy controls, but also between people with this pain who would benefit from a spinal-cord stimulator and those who wouldn’t2. The algorithm had an of accuracy almost 80%, Saab says, showing that even a simple AI can outperform conventional statistics for the task. “Basic machine learning is picking up signals that classic methods and the human eye are not capable of detecting.”
Sensory disturbances
Vollert has seen similar results from applying basic machine learning to an approach called sensory phenotyping. He has used this technique with people experiencing neuropathic pain, which arises from nerve damage. The idea is to assess the broad changes to the sensory system that typically accompany pain. “With a strong headache, for example, many people get very sensitized to sounds or smells,” says Vollert.
Using machine learning to analyse people’s sensitivity to stimuli such as pinpricks, heat, touch and vibration, Vollert and his team were able to group people into three distinct subtypes that were linked to a hypothesis about neuropathic pain mechanisms3.
Being able to categorize people experiencing pain into subgroups could not only help physicians to tailor treatments, but also lead to new targeted pain drugs. The team’s sensory phenotyping method has been approved by the European Medicines Agency for use in human clinical trials. “A company recently used this kind of stratification and found that a drug that previously failed in a trial of all neuropathic pain was successful in a trial of the subgroup that we predicted it would be successful in,” Vollert says. Indeed, he contends, a host of once-promising pain therapeutics that failed late-stage clinical trials might be revived through this biomarker-based stratification route4.
Researchers looking for pain biomarkers have used a range of methods, including functional magnetic resonance imaging (fMRI) brain scans, genomics and proteomics. The turning point for fMRI came in the 2000s with the development of ‘resting state’ fMRI, which scans brain activity in people when they are relaxed and not engaged in any activity or task. “When we compared these resting-state networks in healthy people and people with chronic pain, we could see abnormalities,” Davis says. “The thinking was, maybe the characteristics of those abnormalities might end up being predictive of treatment response.”
Early results were promising. “In 2012, when I first published work using structural brain imaging to classify the presence or absence of lower back pain, we got 76% accuracy,” says Mackey5. Progress then stalled. “Since then, the accuracy hasn’t got much better,” he says.
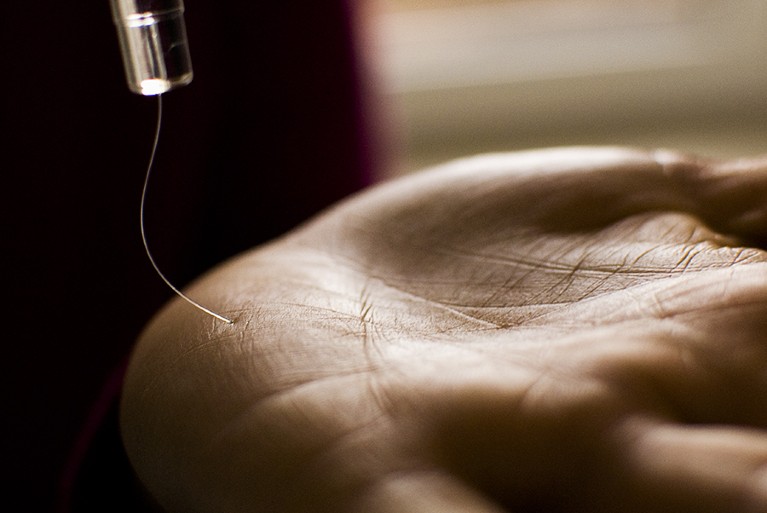
A Von Frey filament is used in sensory phenotype tests to determine pain thresholds.Credit: Christoph Maier
It’s a pattern that has repeated across several methods for measuring pain. “We have all had some form of success,” says Vollert, “but we are all increasingly recognizing the limitations to each of our methods, and finding that they do not explain as much as we would want.”
By pooling data from multiple biomarker techniques, however, a stronger signal should emerge. “Where the field is going is to bring all these data sets together,” says Vollert, who is currently participating in two such efforts in Europe: the IMI-PainCare Biopain consortium led by Rolf-Detlef Treede, a neuroscientist at the University of Heidelberg in Mannheim, Germany, and Painstorm, led by David Bennett, a neurologist at the University of Oxford, UK. “To build really good models, we need prospective data sets that collect all kinds of marker information in parallel from the same patients,” Vollert says. “That’s the kind of data set that we’re generating at the moment.”
If enough people can be included, biomarkers of pain subtypes should emerge that are predictive of treatment response. In the pain clinic, each person’s pain biomarkers could then be compared against these patterns, guiding clinicians to the most effective treatments in each case.
In the United States, Mackey and his colleagues developed the Collaborative Health Outcomes Information Registry (CHOIR), a questionnaire-based digital-health platform. Since it launched in 2012, CHOIR has enabled pain clinicians to collect high-quality pain data in a standardized form from individuals. The platform, which tracks how people respond to each medication they try, has been adopted by clinics across the United States and beyond. Later this year, it will relaunch with the capability to include biomarker data in addition to its current offerings of demographics and symptom patterns. “The idea is to look at a person as holistically as possible, to capture their experience of pain and then to use that information to build models that may predict treatment response,” Mackey says.
Hidden patterns
With multimodal data in hand, advanced AI tools that are more powerful than the simple machine-learning models previously deployed will be crucial to categorizing people into subgroups and treatment selection, says Vollert. “We are working with machine-learning specialists to find models that go much deeper into these huge, rich data sets,” he says.
The latest advanced AI models — including ChatGPT and the AlphaFold protein folding prediction tool — use a machine-learning approach called deep learning neural networks. These models take an input data set and start to make connections between the data points, to generate their output. The goal is that combining deep learning neural networks with big new data sets will enable more fine-grained patient stratification and reach accuracies significantly higher than 80%.
Preliminary findings suggest that advanced deep-learning AI could be transformative for accurate pain biomarker identification, says Mackey. “It’s not published yet, but we’ve just been using some really advanced AI techniques on UK Biobank structural imaging data from a highly heterogeneous population with back pain, and we’re getting classification accuracy around 90%.” The next step — which efforts such as the expanded CHOIR platform are intended to enable — is to link treatment outcomes to this new-found capability to accurately and objectively classify people with pain into subgroups.
One caveat is that these advanced AI techniques apply non-interpretable models to biomarker data analysis. “In my early work with machine learning, I could tell you exactly what brain regions were contributing to the model,” says Mackey. “With the new and improved versions, we can get incredible accuracy, but I can’t tell you what’s driving it.”
Non-interpretable modelling presents a problem for pain-medicine practitioners, Mackey says. “As clinicians, we want to understand the basis of a medical recommendation and not just blindly take the word of a machine — because what if the machine is wrong?”
More from Nature Outlooks
Even given this opacity, physicians could still consider non-interpretable models’ output as one factor in their decision-making process for treatments, Mackey says. In the meantime, the next generation of AI models that are currently in development might show their work more readily. “AI research is moving towards models that are interpretable, but which approach the accuracies of these deep learning, non-interpretable models,” he says.
Personalized pain medicine doesn’t need to be perfect for it to offer a major improvement over today’s chronic-pain treatment. “Right now, as physicians,” Mackey says, “many times we’re doing a mental coin toss between possible treatments.” Even reaching an individual treatment prediction accuracy of 70–80% could reduce the frustration that a person feels and improve their quality of life, he says.
It is going to take a lot of effort and resources to build the big multimodal pain biomarker data sets that are required to reach this point. But, prescribing an effective pain treatment from the start for each person is likely to be a much more cost-effective investment than chasing new pain drugs, Russo argues. “You are never going to get that by having the world’s best super-duper drug, because there’s too much heterogeneity in human pain for that to be ever possible,” he says. “But it may be possible to predictively say, of these 12 good existing drugs, I can now reject 11 of them and select the one that will work for the patient in front of me.”