ITAI YANAI: Exploring human systems with cellular maps
When early European naturalists travelled to South America, they revelled in its unfamiliar geography and studied its exuberant life forms. Although they were not driven by any specific hypothesis, their immersion in this environment led them to conceive a view of nature — one that seemed harmoniously interconnected. A similar spirit of exploration has led the Human Cell Atlas (HCA) consortium1 to document the human body at the level of individual cells, by creating atlases of coherent systems such as the gastrointestinal tract, the lymphatic organ the thymus and cell-based organoid models of the brain.
The Human Cell Atlas: towards a first draft atlas
The atlases rely heavily on the characterization of many individual cells and their RNA transcripts (their transcriptomes), owing to both technological developments and a key biological insight. First, over the past ten years, technology has scaled up to allow scientists to capture the transcriptomes of many thousands of cells in a single experiment. Second, the transcriptome — which might be called the genome’s first observable trait, or phenotype — is able to capture cell types and cell states, as revealed over the past 30 years of research.
One challenging aspect of creating an atlas is to consider the multiple relevant biological axes that it must comprise, principally among them time (developmental and ageing), space (tissue organization) and disease state. The atlases mentioned focus on these axes to different degrees, as appropriate for their system. Their true novelty might be that they integrate data sets and have led to a level of completeness that can now be mined for insights.
Read the paper: Single-cell integration reveals metaplasia in inflammatory gut diseases
The gastrointestinal tract atlas by Oliver et al.2 spans from the tissue of the mouth through to the oesophagus, the stomach, intestines and the colon. Many previous data sets have been created, but the present atlas deftly integrates these — using an innovative computational approach — into a large-scale atlas of 1.1 million cells, along with annotations of the resident cell types and states. On top of this atlas, the authors also ‘layered’ gastrointestinal data sets from individuals with inflammatory diseases that affect the digestive system, including coeliac disease and Crohn’s disease. Intestinal inflammation can cause cells to undergo metaplasia, a shift from one cell type to another. By analysing the layered data sets, the authors inferred the origin of these metaplastic cells by comparing them with stem cells in the atlas. This insight highlights the benefit of the atlas’s completeness, which allows for the comparison of disease states in one organ with the normal states of cells in different organs.
Read the paper: An integrated transcriptomic cell atlas of human neural organoids
Although the brain is intensely studied, organoids have become a powerfully tractable model for functional analysis. The human neural organoid cell atlas described by He et al.3 is built on 1.7 million cells by integrating 36 single-cell RNA-sequencing data sets, generated with 26 protocols for producing organoids from cultured cells. The atlas is already shedding light on the crucial question of how faithfully organoids capture aspects of the developing brain. The authors found a correspondence between the length of time the organoids were in culture and the developmental stages that they resemble in the human brain: in the first three months of culture, the organoid resembles the cellular state of the fetal brain during the first trimester of pregnancy, whereas in the next three months, it resembles the second trimester. But the authors found an intriguing limit to this correspondence. The diversification in neuronal cell types that occurs with development did not continue in the organoid, and the fetal brain during the last trimester of pregnancy was not captured — leaving an open question about which required signals or other features are missing from organoid models.
Read the paper: A spatial human thymus cell atlas mapped to a continuous tissue axis
Yayon et al.4 created a map of the thymus, a lymphoid organ that produces immune cells, in its early fetal development and early postnatal stages. Using the spatial dimension, the authors conceived of a ‘common coordinate framework’ to mathematically map the tissue. This model of the axis between the outer part of the thymus and its centre (the cortico-medullary axis) allows for a deeper understanding of tissue organization and comparison of the organ both in and between individuals. It will be interesting to study how this atlas extends to other stages of life, such as old age.
What do researchers ultimately require from an atlas? As further data modalities — such as single-cell proteomics and metabolomics — become ready for the prime time, it will be crucial to update the atlases. Collectively, the atlases have the potential to constitute a resource that others might be inspired to explore and compare with other biological contexts, such as different species and rare diseases. Researchers might then discover aspects of the human body that cannot yet be imagined, just as the work of the next generation of naturalists led to a radically new — and evolutionary — view of nature.
SIMON HAAS: Cartography of human development at scale
During embryonic and fetal development, the coordinated self-organization of continuously differentiating cells over space and time directs the formation of tissues, the development of organs and the establishment of body functions5,6. Owing to the immense complexity of these processes, scientists’ understanding of the molecular and cellular mechanisms that underlie such developmental events, particularly in humans, remains limited.
The advent of technologies that enable the systematic mapping of molecular features across single cells and tissues — including their genomic, transcriptomic and epigenomic (modifications to DNA and histone proteins that regulate gene expression) states — has dramatically advanced researchers’ understanding of complex cellular systems7,8. Through multidisciplinary efforts, such as the HCA initiative1, teams around the world have started to decode cellular identities and the architectural principles of tissues and organs with unprecedented detail and resolution.
Read the paper: A prenatal skin atlas reveals immune regulation of human skin morphogenesis
As part of the developmental branch of the HCA, Gopee et al.9 and To et al.10 have shed light on the embryonic and fetal development of the human skin and skeleton. Using advances in scaling single-cell technologies, improved spatially resolved mapping techniques and powerful analytical frameworks, these studies provide valuable insights into early human development. In both studies, the researchers generated comprehensive maps detailing the dynamic changes in cellular states as developmental progenitor cells progress through continuous differentiation, lineage specification and cell-type specialization. They also mapped the spatial location of these cells and inferred potential intercellular signalling cues that could drive the formation of organs and tissue function.
Read the paper: A multi-omic atlas of human embryonic skeletal development
To and colleagues explored the embryonic and fetal development of part of the skull and joints of the limbs 5–11 weeks after conception. Through the simultaneous mapping of transcriptomic and epigenomic profiles of single cells, they identified key gene-regulatory networks that direct the commitment of cells to chondrogenic (cartilage-forming) and osteogenic (bone-forming) lineages. The authors inferred probable lineage relationships along differentiation pathways and propose how cellular crosstalk might guide the formation of bone, identifying a potential key role for interactions with the vascular system. Using an elegant approach, the authors further integrated data derived from genome-wide association studies with their single-cell analysis to identify developmental cell states that are potentially linked to complex traits of the adult skeleton, such as osteoarthritis, a disease that affects the joints.
Similarly, Gopee and colleagues present a comprehensive cellular atlas of skin development spanning 7–17 weeks after conception. Using a combination of single-cell and spatial transcriptomic technologies, the authors mapped dynamic changes in cell states and detail how these cells organize to form developmental structures and interact in microanatomical skin niches. Their findings highlight the unexpectedly diverse role of immune cells in coordinating developmental processes, particularly the involvement of macrophages in the formation of blood vessels by endothelial cells. This was further validated through an innovative organoid system that recapitulates key aspects of skin development.
Both studies provide an invaluable foundation for understanding early developmental processes in humans. However, findings related to cellular relationships, cellular crosstalk and the establishment of tissue architecture are mostly based on inferences from transcriptomic data, and should therefore be considered as hypothesis-generating discoveries. Future advances in organoid technologies and other mechanistic models will be essential to validate and expand these findings.
CHRISTOPH LIPPERT & HELENE KRETZMER: Machine-learning innovations for single-cell analysis
Almost a decade ago, the HCA consortium1 initiated an ambitious project to chart a comprehensive map of all human cell types across tissues and throughout embryonic and adult stages of life (Fig. 1). Mapping such complexity is challenging11 because of shifting technologies, variability between experiments and inherent noise in sparse data that results from having small amounts of biological material to work with. The continuous transition between cell states and the increase in ‘omics’ data modalities (including transcriptomics, epigenomics, proteomics and metabolomics) further complicate standardized cell-type identification and classification, which often need manual adjustments. If these issues are not handled adequately, biological interpretations can be compromised, highlighting the need for techniques that robustly correct errors and integrate data from different modalities11–13. As a result, the field of single-cell multi-omics has seen a surge in methods that tackle specific challenges14–17, but there is still scope to further standardize models and refine their complexity for optimal performance. Three machine-learning algorithms, developed by researchers in the HCA consortium, address cell annotation and data integration.
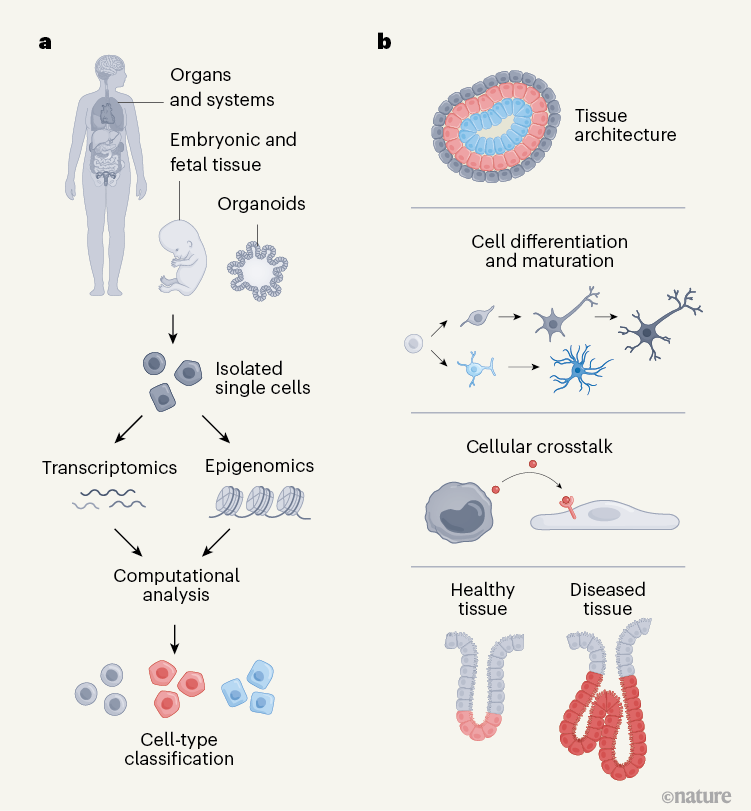
Figure 1 | Human cellular atlases. a, The Human Cell Atlas project aims to create cellular maps of human organs and tissues throughout life, and in health and disease. Cells are isolated from tissues during different stages of development and from cell-based organ models (organoids). Cell types and states can be captured using single-cell profiling techniques, mainly transcriptomics (using RNA transcripts to examine gene expression) but also other methods such as epigenomics (examining regulation of gene expression by assessing modifications to DNA and histone proteins). Sophisticated computational analyses are used to classify cell types and integrate information from different data types. b, Cellular atlases can be used to make inferences about human biology: spatial transcriptomics enables cells of defined type to be mapped to the tissue from which they originated, so that tissue architecture can be examined; cell differentiation and maturation during developmental processes can be traced; interactions between cells of different types can be inferred; and comparisons can be made between healthy and diseased tissue.
Ergen et al.18 introduce popV, a model for classifying cell types that focuses on transferring cell-type labels from annotated atlases to unannotated data sets. The popV model is an ensemble model, which means that it combines classification predictions from existing models to produce both cell-type labels and uncertainty scores based on the degree of disagreement between the underlying tools. This approach highlights ambiguous cases, which reduces manual review and draws attention to cell populations that are challenging to classify. This feature enhances the interpretability of results and streamlines the overall annotation process by reducing the load on researchers, making popV adaptable to future models.
Fischer et al.19 present scTab, a deep-learning model tailored to annotating cell types across various tissues using single-cell RNA-sequencing data. Recognizing the limitations of conventional machine learning in handling large, diverse data sets, the developers of scTab introduce a data-augmentation approach for single-cell sequencing data to increase the size of the training data set, enabling generalizations to be made across tissues. Fischer et al. demonstrate that complex nonlinear models outperform simpler linear ones in cell-type classification, especially when trained on extensive data sets. The potential of scTab lies in promoting standardized cell labels and encouraging a research-community-wide consensus in cell-type nomenclature.
Cell-level reference maps for the human body take shape
MultiDGD, described by Schuster et al.20, tackles integrating multimodal data, such as gene expression and the accessibility of chromatin (the packaged form of DNA) to transcriptional machinery, using a ‘deep latent variable’ model. This type of machine learning uses hidden variables to learn complex patterns — in this case, multiDGD learns optimal hidden-variable representations that are shared across multiple data modalities (transcriptomic and epigenomic), without the need to pre-define important features. By incorporating information about potentially confounding variables, such as inconsistencies between samples, multiDGD enables post-hoc data integration across data sets, making it suitable for multi-omics studies, in which data were gathered from different sources. This model’s clustering of shared representations improves the alignment of multimodal data, enabling associations between genes and regulatory regions of the genome to be mapped — an essential step in understanding gene-regulatory networks.
Together, popV and scTab lead efforts in standardized annotation and consensus-building, whereas multiDGD opens up avenues for data integration across complex multimodal data sets.
Single-cell sequencing is a rapidly evolving field in which technological advances continuously reshape scientists’ understanding and definitions of cell identities. Atlases such as the HCA will probably remain as ‘living resources’, adapting to incorporate new data and discoveries. Machine-learning methods, including those introduced by these three groups, offer innovative frameworks for integrating and analysing this fast-growing data source. However, as with any field that is dependent on rapidly changing data, models trained on existing data sets will have limited lifespans; they are finely tuned to the specific data sets used in training and might require adaptation as data emerges and data-collection biases evolve.
This does not lessen the impact of these methods, but rather highlights the field’s rapid pace and the importance of innovation. To meet this growth, future research is likely to emphasize adaptable and interoperable solutions. These methods contribute valuable foundations for future advancements, paving the way for even more adaptable and scalable models for single-cell, multi-omics data.