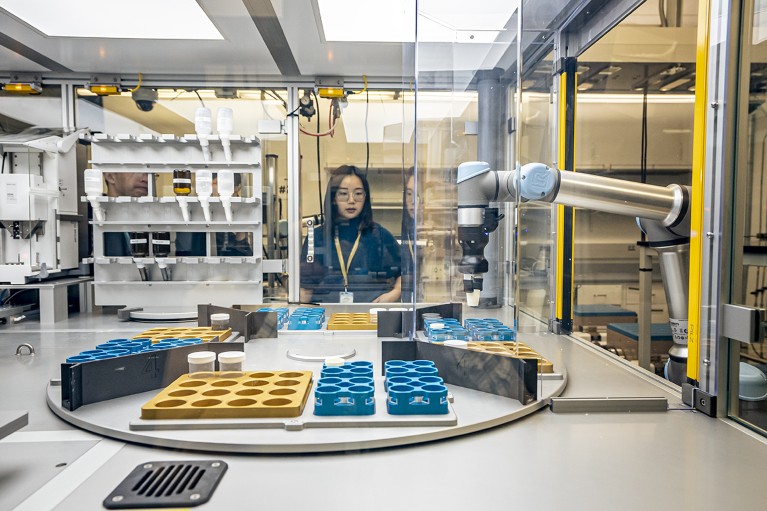
Researcher Yan Zeng looks over the machinery at the A-Lab, a fully automated laboratory at the Lawrence Berkeley National Laboratory in California.Credit: Marilyn Sargent/Berkeley Lab
Stephan Noack’s official title is bioprocess engineer. In simple terms, he is a problem-solver. His colleagues at the Jülich Research Centre in Germany knock on his office door armed with some of their thorniest questions about the process of coaxing bacteria, algae and other microbes into mass-producing valuable chemicals, such as ethanol and amino acids. Optimizing such processes requires making tiny adjustments to several variables, including the microbes’ food source and growing temperature. It’s trial and error — mostly error. “During the set-up of these workflows, a lot of failure happens,” Noack says.
Even the most efficient laboratories, with an ample amount of students to conduct the trials, can fail to complete the lengthy, laborious process. “It was a huge bottleneck,” he says. Noack and his engineers have therefore been turning to robotics and automation to speed up the process of growing microorganisms on plates of gelatinous agar. By combining a range of equipment, from robotic arms to liquid handlers, researchers have been able to swap out large single plates of agar for ones containing 96 or 384 tiny wells. This has increased throughput nearly 100-fold according to Noack.
Nature Outlook: Robotics and artificial intelligence
Although they are common in large industrial research facilities, robotics and automation have only begun to trickle down into smaller academic labs in the past five years, says Ian Holland, a postdoctoral researcher at the University of Edinburgh, UK. Historically, he says, academia has relied on large populations of students and postdocs to do the time-consuming work. But with scientific advances requiring ever-increasing amounts of data generation and analysis, lab workers can’t work quickly enough. But robots can.
The advances include robotic arms that can pipette more accurately than can human scientists1 and fully automated ‘cloud’ labs that experimenters can access online and command a robot workforce to perform their instructions from anywhere in the world2. Researchers who are leaning towards automation hope that the shift will decrease cost, save time and generate fewer errors while improving reproducibility.
But these changes don’t come without challenges. Scientists need a deep understanding of their experiments to program machinery and to prevent the propagation of errors. The equipment can be expensive and require hours of labour to fix and maintain. If done correctly, however, laboratory automation can transform science, according to Dennis Knobbe, a roboticist at the Technical University of Munich in Germany. “It’s not about excluding the human from these processes,” Knobbe says. “It’s instead about using robotics to enhance researchers’ capabilities.”
Rise of the machines
In 2012, Matheus Carvalho, a research technician and fisheries biologist at the Southern Cross University in Lismore, Australia, encountered AutoIt, a programming language originally created for automating Microsoft Windows tasks. Around the same time, he came across a toy robotic arm that could be controlled through a computer. Carvalho reasoned that if he could combine the toy robot with AutoIt, he could automate some of his tedious sampling tasks in the lab. Although the first robotic arm broke almost immediately, Carvalho convinced his supervisor to purchase a higher-quality, second-hand arm, which was built into an automated sampling machine that continues to operate more than a decade later. Carvalho was quickly sold on the idea of laboratory automation, which is the topic of a book he published in 2017.
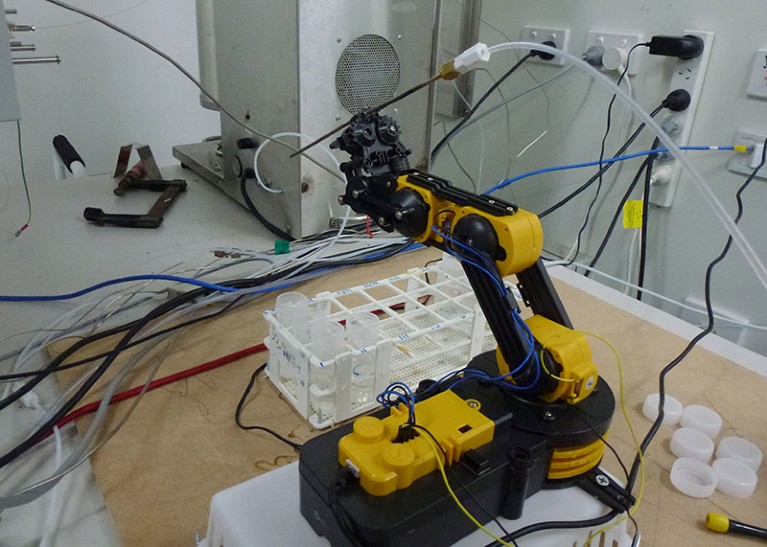
Matheus Carvalho at the Southern Cross University in Lismore, Australia, created an automated sampling machine using a reprogrammed toy robotic arm.Credit: Matheus Carvalho de Carvalho
He aimed to automate more lab procedures without precluding human involvement. His lab used non-radioactive isotopes to understand organic material in water samples — a process that requires weighing and measuring tiny amounts of powders, often to a fraction of a milligram. Every powder they tested had a different grain size and texture, which made it impossible to program a robot to measure out all the samples. Instead, Carvalho devised a protocol that allowed people and machines to each do what they were best at: a human lab technician weighed out the powder samples, and a small, mobile robot was programmed to retrieve containers and calibrate the scales. “It’s better to automate what is easy but leave the hard parts for us humans,” Carvalho says.
In the 2010s, Dina Zielinski, who was then a technician at the Whitehead Institute in Cambridge, Massachusetts, faced similar challenges with automation while working on a different type of test. She wanted to sequence tissue samples from people with Parkinson’s disease to understand the genes contributing to the condition. The job required pipetting — a lot of pipetting. Zielinski saw the task in front of her as a fast track to repetitive strain injury.
“Molecular biology essentially entails combining minuscule clear volumes with other miniscule clear volumes,” Zielinski says. “If you didn’t combine the right tiny volumes, you would have wasted a ton of money on sequencing.”
Even worse, she says, these samples were rare and hard to obtain. Yaniv Erlich, who was then a principal investigator, and his late collaborator Susan Lindquist, a biomedical researcher at the Whitehead Institute, began investigating various robotics, including automated liquid handlers, to speed up the process and to save Zielinski’s hands from injury. But none of the robots they investigated could provide both the precision and flexibility that the lab needed. So, Zielinski, Lindquist and Erlich, who is now chief executive of Eleven Therapeutics in Cambridge, UK, decided to build something different.
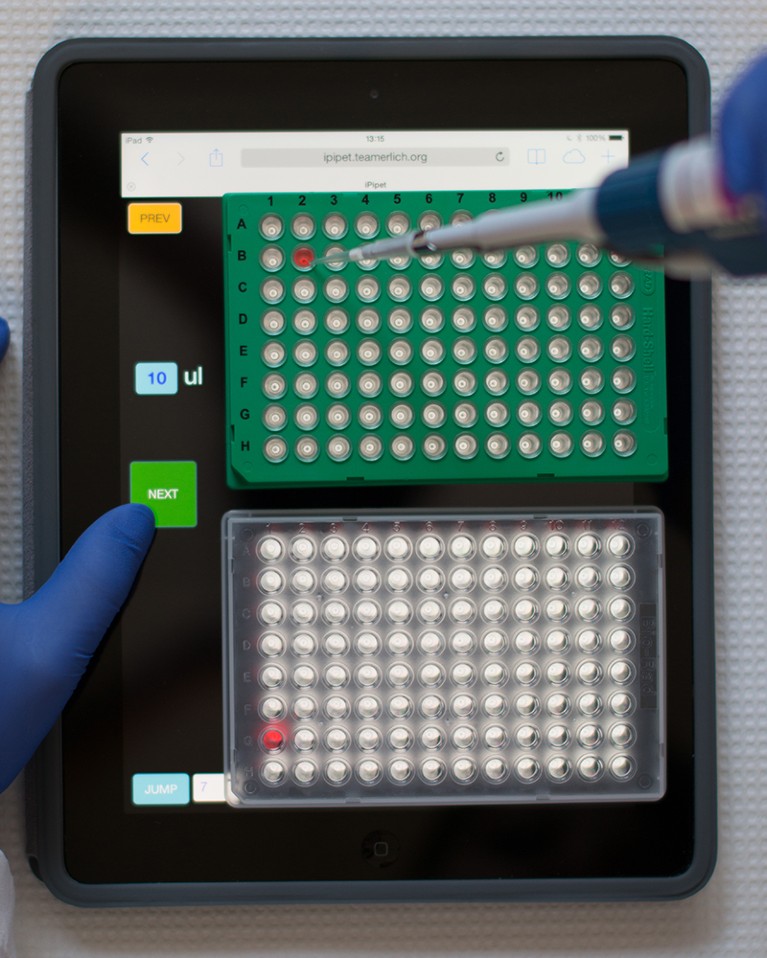
The iPipet app can be used to illuminate sections of a 96-well plate and help researchers to ensure they combine the correct samples.Credit: Dina Zielinski
The idea they came up with didn’t handle the pipetting itself. Instead, the team built an iPad app that users could program to help them pipette the correct samples into the correct position. The iPipet app illuminates sections of 96- or 384-well plates to enable a scientist to ensure they combine the correct samples3. When Zielinski pitted a researcher using iPipet against a top-of-the-line robot, the app-assisted human was the clear winner. “The error was much lower with human pipetting than with the liquid-handling robot,” she says.
Mind the gap
What makes efforts such as these so trailblazing isn’t their complexity but rather their simplicity. The goal is to find a middle ground between the expensive instruments that can perform every aspect of an experiment and the labour of a single student performing all their tasks manually, Holland says. Ideally, such technology would make it possible for researchers to spend time planning experiments and analysing results instead of pipetting samples.
“If automation can take some of the load off you, you can do more things and be a better researcher,” Holland says. And the academic environment is well-suited for this melding of human and machine. “You’ve got engineering students looking for projects and we’ve got biologists who have problems that need solving.”
However, the changes come at a cost says Holland. Since the dawn of the industrial revolution, people have invested time, resources and money into developing machinery to make products more quickly and cheaply. In commercial settings the benefits were clear, says Holland — investment in automation paid off because it allowed production of more commodities with low labour costs.
Academia is different. Industry focuses on profit, whereas academic labs place a greater emphasis on training the next generation of scientists and producing knowledge. A steady flow of students who are willing to work long hours — some of whom have their own grants and stipends as salary — means that labour costs aren’t as important. What’s more, the focus on teaching and training means that many scientists have conventionally seen automation as anathema to their mission as educators.
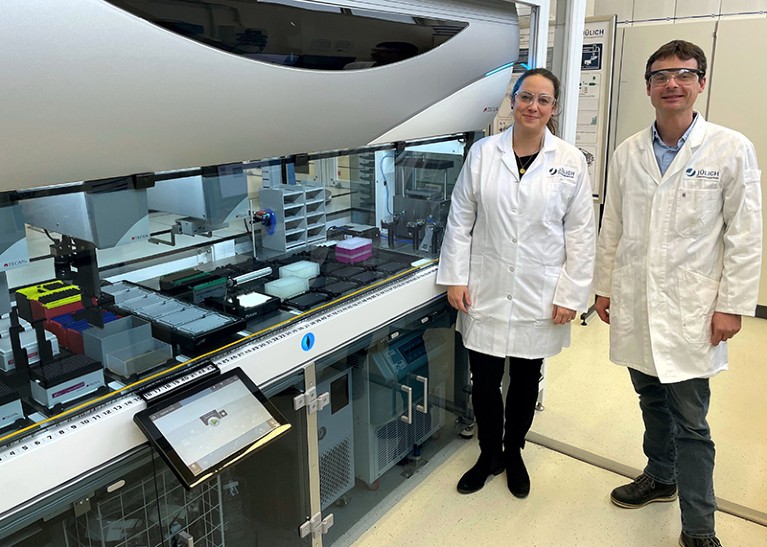
Postdoc researcher Julia Tenhaef and bioprocess engineer Stephan Noack at the Jülich Research Centre in Germany use an automated laboratory system called the AutoBioTech platform. Credit: Stephan NoackCredit: Stephan Noack
“In academia, you could spend US$100,000 on this machine, but it’s only going to make your output a bit faster,” says Holland. “That’s a lot harder to justify.” As a result, many academic labs have much less robotic equipment than do commercial and industrial labs — something Holland refers to as the automation gap4.
Joshua Pearce faced down these technological costs when he founded his lab at Michigan Technological University in Houghton in the mid-2000s. Now an engineer at Western University in London, Canada, Pearce was developing methods to build better photovoltaic systems to generate electricity from sunlight. He wanted to improve solar cells’ ability to absorb different wavelengths of light, but the automated filter wheel changer, which adjusted the wavelengths on his custom-built machine, broke. The replacement was $2,500 (an exorbitant price for a simple part) and had a five-month lead time.
Pearce realized that he was at a university filled with budding engineers, so he hired some students to help him 3D print the necessary components. What resulted was a bespoke device crafted entirely from open-source hardware and software that cost $50 and did exactly what Pearce needed it to. “It was something that wasn’t available on the market,” Pearce says. “You can make really high-end equipment, exactly what you want, and do it fairly easily for extremely low cost.”
With his equipment that could automatically adjust light wavelengths for his tests, Pearce began campaigning about the potential of open-source design as a cost-effective way to reap the benefits of lab automation5. He is now editor-in-chief of the journal HardwareX, a publication that allows researchers to share their code and blueprints — while also helping to bolster their CVs and tenure qualifications.
Pearce’s experiences challenge the idea that investing in automation hampers a scientist’s ability to train students, along with the opinion that robotics are prohibitively expensive.
Plain and simple
When it comes to the future of lab robotics, Knobbe thinks that inventions such as those created by Pearce, Carvalho and Zielinski will be key: modular, multipurpose and budget-friendly. “We don’t want to just build a huge machine, like an encapsulated system,” Knobbe says. “We want to integrate these robotic systems into everyday laboratories.”
He also imagines fully fledged robotic lab assistants that can perform basic experimental tasks with minimal supervision. Although this technology is nowhere near ready, Knobbe says, he thinks researchers will be able to deploy modular automated systems that can interact with each other and be controlled by a robotic assistant in the next ten years. One of the biggest challenges will be balancing robustness, flexibility, the ability to detect errors and asking for help.
Building or buying a top-of-the-line machine that only does pipetting would force lab technicians to work around the machine. Knobbe wanted a robot that would work with his team, follow basic commands and scan the environment for obstacles. He is therefore building a robotic pipette with finger-like appendages. Early testing shows that this machine has met industry standards, he says.
Although reducing variability and mistakes has long been one of the selling points of robotics and automation, Knobbe says that robots can also propagate errors1,4. Knobbe also speculates that robots might create types of catastrophic failure.
A cautionary tale emerged in November last year, when a team of scientists from Google DeepMind in London, the University of California, Berkeley, and the Lawrence Berkeley National Laboratory in California teamed up to predict nearly 400,000 new compounds using artificial intelligence (AI) and then to synthesize these compounds in a fully automated laboratory, called A-Lab. The project was an endeavour to identify new high-performance, low-cost materials by automating both the physical synthesis of compounds and their subsequent analysis. A resulting Nature paper6 seemed to showcase the benefits of automation.
“It was a high-risk, high-reward project,” says co-author Yan Zeng, a former researcher at the Lawrence Berkeley National Laboratory who started her own lab at Florida State University in Tallahassee this year. “It was a little bit crazy, to be fully automated.”
Several weeks later, however, some scientists began raising questions about the AI’s ability to predict truly new materials. What seemed to be new in the computer’s modelling might have been different versions of known compounds. “This paper did not at all live up to its claims,” says Leslie Schoop, a chemist at Princeton University in New Jersey.
To Zeng, however, the study was as much about the process — demonstrating how such a system could be built, operated and used by materials scientists — as it was about the results. In fact, Zeng says, the robotic synthesis aspects of the study performed exactly as expected. She concedes that the initial programming steps took months and required a team of technicians to troubleshoot the process. But they quickly recouped the lost time as the robots required minimal human contact.
Zeng is now working to automate parts of her lab in Florida. Her first target is hydrothermal synthesis — a process that requires high temperatures and pressurized tubes. It’s a complex project, but her time at Berkeley gave her valuable experience in breaking down complex robotics into more manageable steps, and she hopes to begin automating this process as she scales up her lab.
Despite the scepticism over A-Lab, she remains optimistic about automation. Robotics could provide the key to future breakthroughs, she says, equipping researchers with the freedom and flexibility to think up the experiments of tomorrow. “This is a rising field, and it’s rising up pretty fast,” says Zeng.