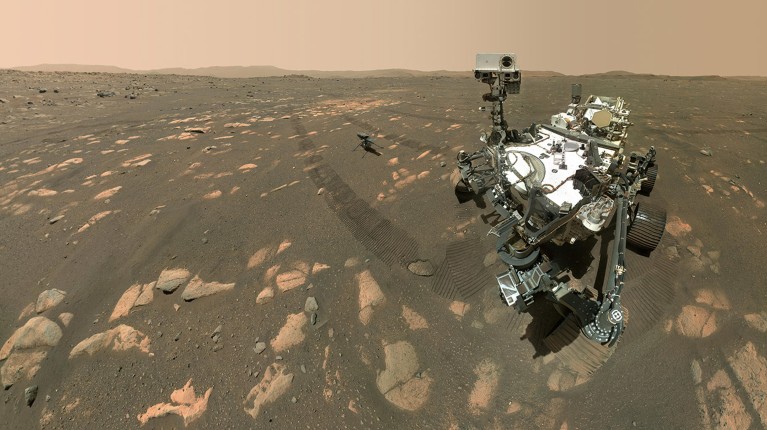
Scientists want to use AI agents to study rock samples retrieved from Mars.Credit: NASA/JPL-Caltech/MSSS
Artificial intelligence (AI) researchers have created a system that can perform autonomous research in astrobiology, the study of the origins of life in the Universe.
AstroAgents comprises eight ‘AI agents’ that analyse data and generate scientific hypotheses. It joins a suite of other AI tools that aim to automate the process of science, from reading the literature to coming up with hypotheses and even writing papers.
Researchers built an ‘AI Scientist’ — what can it do?
The tool’s creators say they will use it to study samples that NASA plans to retrieve from Mars. The agents will help to determine whether the samples harbour organic molecules that indicate the presence of past or present life. The researchers presented AstroAgents on 27 April at the International Conference on Learning Representations in Singapore.
“It’s helping us build a better understanding of how molecules form in space, how molecules form from life on Earth and how they’re preserved — and then which specific signs should we be searching for,” says astrobiologist Denise Buckner at the NASA Goddard Space Flight Center in Greenbelt, Maryland, who co-authored a preprint describing AstroAgents1.
AI agents
The tool is an example of ‘agentic AI’ systems. These are typically based on large language models (LLMs) and are designed to be more-active participants than conventional AI tools, deciding what needs to be done and how to do it, evaluating outcomes and adapting in response. Their emergence has prompted lively debate about whether agentic AI can come up with truly original scientific ideas, and how novelty should even be defined.
One of the most prominent examples is Google’s AI ‘co-scientist’, which was released in February and has searched for potential treatments for liver disease and suggested how antimicrobial resistance arises. Applying agentic AI to astrobiology is new, says astrobiologist Michael Wong at Carnegie Science’s Earth and Planets Laboratory in Washington DC.
Mars rover makes epic climb to explore some of the oldest rocks in the Solar System
To specify the behaviours of the agents, the researchers feed different prompts to an LLM. For example, a ‘data analyst’ is told to identify important patterns in data, a ‘planner’ to decide what to delegate to other ‘scientist’ agents for further research and hypothesis generation, and a ‘critic’ to evaluate the hypotheses and suggest improvements to the data analyst, which then kicks off another round of the process.
The way AstroAgents splits the hypothesis generation up between multiple specialist agents is innovative, says co-author Amirali Aghazadeh, a computer scientist at the Georgia Institute of Technology in Atlanta.
“We realized that because of the complexity of the data, it’s better for the agent to assign multiple tasks to multiple ‘scientists’,” he says. It’s up to the planner to decide what each scientist agent will study, and it does this on its own. “It’s kind of the magic of the system,” he says.
Heaps of hypotheses
The research team experimented with using two LLMs to power AstroAgents — Claude Sonnet 3.5 and Gemini 2.0 Flash. They fed each system mass-spectrometry data for eight meteorites and ten soil samples taken from locations around Earth, including Antarctica and the Atacama Desert in Chile, and carried out ten rounds of refinement.
Astrobiology: Hunting aliens