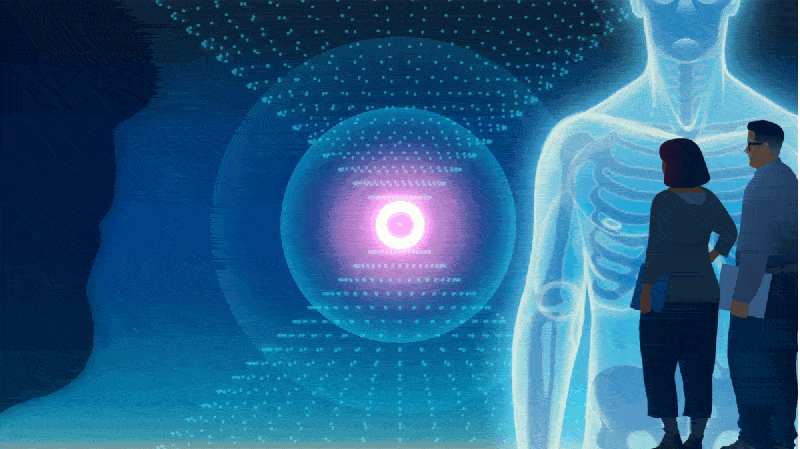
Credit: Sam Chivers
As she listened to her patient speak, laryngologist Yael Bensoussan knew immediately what was wrong with him.
Part of Nature Outlook: Medical diagnostics
The man’s daughter had suggested he see Bensoussan because his voice sounded weak. In Bensoussan’s office at the University of South Florida (USF) in Tampa, she could hear the problem. “I said to him, ‘You have water in your lungs. You have a cardiorespiratory disease.’ And he said, ‘How do you know?’” recounts Bensoussan, who is director of the Voice Center at USF Health. It was because he couldn’t hold a note for more than a few seconds without running out of breath, a sign that his lungs weren’t producing enough air.
She sent him to the emergency department, which found he had a heart condition that had led to pulmonary oedema, resulting in a litre of water in his lungs. Bensoussan says that her training and years of experience make it fairly easy to notice the effects that health issues have on people’s voices even without medical instruments, such as a laryngoscope, but she knows that this is not the case for most people. So she and other researchers are training artificial intelligence (AI) models to listen for signs of various conditions in the sounds that people make.
“Usually when I come in the room, I know what my patient has before putting the scope in,” Bensoussan says. A neurologist can tell who has motor neuron disease (amyotrophic lateral sclerosis) by listening to their voice. But with a boost from AI, she says, non-specialists would have the same ability, and it might even help to identify conditions that she cannot because the signs are too subtle.
Scientists are looking for voice biomarkers for a variety of health conditions, including diabetes and coronary artery disease, as well as menopause. The voice, they think, carries signatures of health and illness, and with the help of now-ubiquitous recording technology and ever-improving AI models, they’re trying to tease out those signatures for screening, earlier diagnosis and remote monitoring of health.
Voice biomarkers could improve telemedicine by providing an easy, non-invasive way to check for various conditions. Individuals could record a snippet of speech on their phones and send it to their physicians for evaluation. Those running clinical trials could increase monitoring of participants without making them travel to a hospital. Physicians might get early warnings of a range of conditions, from Alzheimer’s disease to COVID-19, and request follow-up tests that could lead to swift treatments.
An intricate instrument
Several parts of the body combine to create a person’s voice. The lungs and larynx produce the sounds. The jaw, lips and tongue form speech. The brain controls the language and the content. Physical and mental conditions that affect any of these can create a voice signature that can be detected, often by the untrained ear but sometimes only by computer analysis.
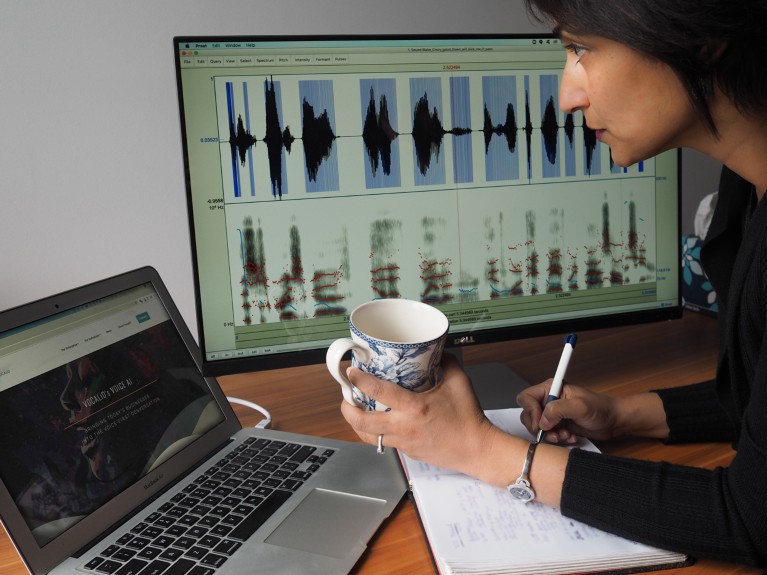
Speech and language pathologist Rupal Patel studies variations in the melody of speech.Credit: Michele Martin
Factors such as muscular control, swelling, hormonal changes and mental status can all affect the quality of a person’s voice, often in ways that are specific to the condition. Menopause lowers oestrogen levels, for instance, which causes tissues to lose water content and collagen. That manifests as shrinkage in the vocal cords, and the voice becomes weaker and rougher. The altered cords vibrate more slowly, which is why the voice drops in pitch post-menopause. Menopause is a normal part of healthy ageing, but voice biomarkers could be used as a way to better understand the changes that lead up to it, or to make decisions about the timing and dosage of hormone replacement therapy. It’s also important to distinguish changes due to menopause from those resulting from other causes.
Parkinson’s disease shows up in the voice as a reduction in the variation of pitch and volume, resulting in a monotone. People with this disease can also lose fine control of the muscles involved in speaking, including the jaw and the tongue, resulting in poorly articulated words. Research has found that speech changes might precede other motor-control deficits by as much as a decade1. AI might be sensitive enough to notice those changes before a clinician can, perhaps prompting physicians to refer people for other tests that could lead to earlier diagnosis and treatment.
Even conditions that might seem unrelated to speech can show up in the voice. Researchers at the Luxembourg Institute of Health used AI to analyse recordings of around 600 individuals, and discovered that the algorithms could detect type 2 diabetes. In a study published in December 2024, AI correctly identified 71% of diabetes cases in men and 66% in women purely by analysing snippets of their recorded speech2. Researchers know that diabetes causes certain vocal changes3, some of which might be caused by swelling as a result of increased levels of glucose4, or by nerve damage from untreated diabetes5. Bensoussan, who has collaborated with one of the study’s co-authors, finds the results impressive. She says that even with her skills, she can’t tell if somebody has diabetes just by listening to them speak.
Voice is an acoustic instrument that’s very sensitive to physiological changes in the body, says Rupal Patel, a speech and language pathologist at Northeastern University in Boston, Massachusetts. “There are all sorts of acoustic characteristics of voice that are measurable,” Patel says. Fluid retention, which can be caused by heart disease, increases the mass of the vocal cords, for instance. This makes them vibrate more slowly, decreasing the pitch of the voice. Heart disease can also cause breathlessness, as speakers struggle to push enough air out of their lungs.
The challenge for scientists is to relate the patterns of the signals that they detect to particular diseases. “To say a breathier voice is someone with heart disease is oversimplifying this, because it’s not just one thing,” Patel says. “It’s usually a combination of multiple cues together that help us differentiate between someone who is healthy, someone who is dehydrated, and someone who has heart disease.”
For instance, a certain percentage of women with Parkinson’s disease are also menopausal. That means signals from both the disease and menopause — and any other conditions that a person might have — need to be teased apart to get an accurate assessment. “No one is a neat Parkinson’s patient,” Patel says. “Most of us have other things that are going on too, and all of these things have an impact on voice.” Although science has identified a number of voice changes due to various conditions, much work remains to be done to work out how to deal with overlapping signals, she says.
Further muddying the water is that the link between physiological changes and voice biomarkers is not always evident. Amir Lerman, a cardiovascular specialist at the Mayo Clinic in Rochester, Minnesota, says that AI can sometimes produce signatures that are good predictors of disease, but that are not easily explained. Lerman and his colleagues asked volunteers to read a prepared text, then used AI to analyse their voices. The AI produced a heat map showing variations in the frequencies of various voice features, and some of the mapped features were more prevalent in people who were known to have coronary artery disease6. “We don’t know for sure what the mechanism is for that,” Lerman says. The team also found voice biomarkers for pulmonary hypertension, in which blood pressure increases in arteries in the lungs and the right side of the heart7, and for heart failure8.
A voice biomarker is unlikely to replace existing tests, but it might be used in conjunction with them. A biomarker for coronary artery disease, for example, could prove to be a good preliminary test before an angiogram, which is invasive and tends to be given to individuals who already show significant signs of disease. Because voice is “so non-intrusive and you could do it at home, it’s going to be, I think, very useful”, Lerman says. It could also be a good way to evaluate a person after treatment. If a voice signature, delivered over the telephone, looks good, the physician might decide that the treatment is working and that the individual doesn’t need to make a trip to the clinic. “We’re not making decisions only because of one algorithm,” Lerman says.
Assessing mental health
Speech is already used, without the assistance of AI, to diagnose mental-health conditions, from depression to Alzheimer’s disease. Physicians ask people to remember and repeat a series of words to test for memory problems. Individuals with depression tend to speak more softly and slowly, and to speak in more negative and absolute terms, than do people without depression910. These factors have more to do with the brain than the vocal cords. Researchers have also found, however, that acoustic features, such as ‘vocal jitter’ caused by the way that the cords vibrate, can also indicate depression11. AI has the potential to extend the use of such markers. “One of the things that these kinds of technologies allow us to do is to think about how we could be measuring people more frequently, less invasively, and longitudinally,” says Peter Foltz, a cognitive scientist at the Institute of Cognitive Science at the University of Colorado Boulder.
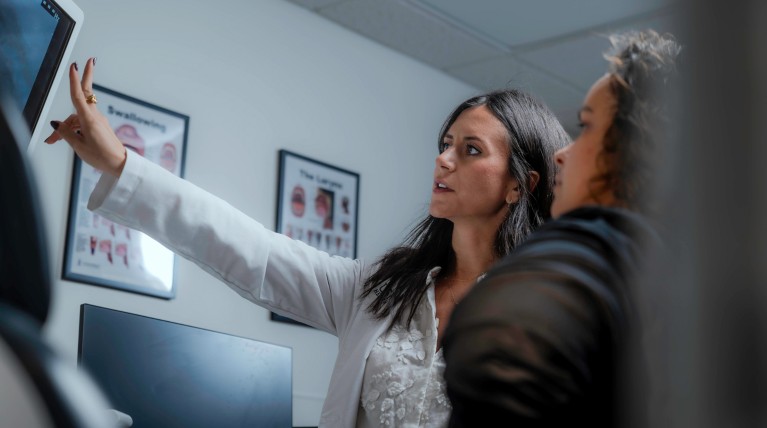
Yael Bensoussan (left) is adept at recognizing how health conditions alter speech.Credit: Andres Faza
Foltz and his colleagues developed an app to assess the mental status of people with psychiatric conditions such as depression and schizophrenia, then used AI to score the results12. Such examinations, which look at factors such as rate, rhythm, volume, tone and amount of speech, are routinely done by clinicians, but there aren’t enough specialists to assess individuals as often as they’d like to. The technology worked well enough that, with further development and validation, it might help clinicians by providing them with frequent measurements of their patients’ mental states. “We’re still at the stage of running these on tens or hundreds of people, and not on the thousands or tens of thousands you would need to be validated,” Foltz says.
Some attempts to diagnose dementia from voice patterns have shown promise. Ioannis Paschalidis, a computing engineer at Boston University in Massachusetts, applied AI to voice recordings of people with mild cognitive impairment and found that he could predict which people would develop Alzheimer’s within six years, with an accuracy of almost 80% (ref. 13). He and his colleagues took recordings of interviews with 166 people in the Framingham Heart Study — a long-running study of cardiovascular health. They knew from the records that 90 of those people with mild cognitive impairment would decline over the 6 years after the recording was made. The AI model identified which people would go on to develop Alzheimer’s, on the basis of an analysis of the content of the speech, not the acoustic features.
The long haul
One challenge with finding voice biomarkers for psychiatric conditions is that a person’s mental state can fluctuate rapidly, in a matter of days or even hours. But collecting enough data to understand the fluctuations, and to see what changes might be predictive of emotional distress, is a long process, says Brita Elvevåg, a psychiatrist at the Arctic University of Norway in Tromsø. “We know that we can hear it in voice when somebody is distressed, but the question is, can we do so before?”, says Elvevåg, who first collaborated with Foltz when she worked at the US National Institute of Mental Health in the 1990s. Her goal is to glean information from AI voice analysis to help predict when someone might be in distress, so that she can try to head it off.
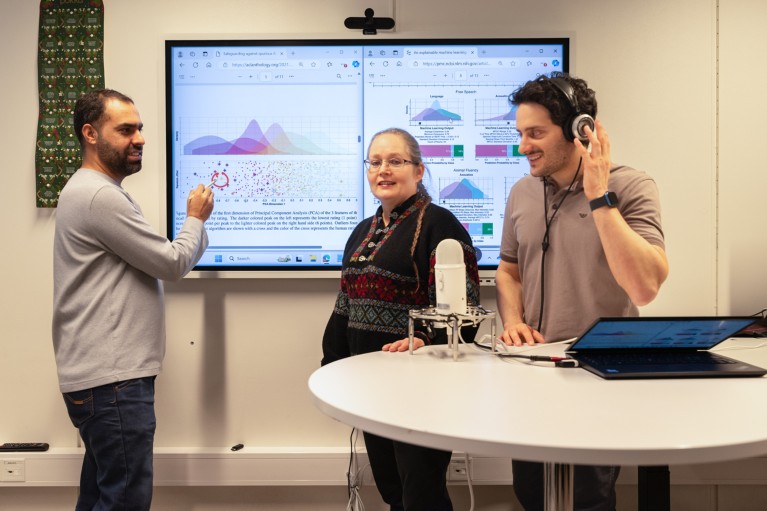
Psychiatrist Brita Elvevåg with postdocs Musarrat Hussain and Enrico Tedeschi.Credit: Enrico Tedeschi
There’s plenty of general psychiatric data collected from thousands of people and sorted by factors such as age, gender and ethnicity to identify how prevalent certain voice signals are in a particular group, but most data are based on observations at a specific point in time. “Now, what we’re trying to do is suddenly to use technology to model, to understand longitudinally how we’ll feel tomorrow, how we’ll respond a year later,” Elvevåg says. “We just don’t have that database.”
In fact, the whole field of voice biomarkers needs more data that monitor how voice changes over time with the conditions that researchers are studying — both to understand how the signals progress with disease and to know what an individual’s baseline state is. Although researchers can identify whether a person’s voice signals match a cohort that is known to have a particular condition, they currently have no way of comparing them with what is normal for that individual, making diagnosis difficult. “Just because someone has reduced pitch, it doesn’t mean that they’re depressed,” Patel says. “We need research methodologies that help us capture more longitudinal data. Because until you have longitudinal data, we don’t know about individual variation.”
There is not yet a large collection of standardized samples that can be used for research — nothing, that is, akin to the data sets that have propelled discoveries in genomics and radiology images. To rectify that, in 2022, Bensoussan teamed up with Olivier Elemento, a physiologist at Weill Cornell Medicine in New York City, to launch the Voice as a Biomarker of Health project. The 4-year, US$14-million project, funded by the US National Institutes of Health, involves researchers at 50 institutions. The aim is to collect voice data of 10,000 people to create a publicly available data set on which to train AI. (NIH funding was in flux as of mid-May, with the administration of President Donald Trump ordering widespread cuts and courts pausing those orders.)
Participants perform 20 voice-related tasks, including reading specific texts, speaking freely in answer to questions, breathing, coughing or enunciating a long ‘e’ sound (as in the ‘e’ sound in ‘feet’). At its halfway point last December, the project issued its first data release: 12,500 recordings of 306 people in the United States and Canada.
The project is also developing methods for the ethical use of voice data. Like any health data, voice recordings can carry private information that a person might not want to disclose. In its initial release, the project did not supply raw recordings, only spectrograms — visual representations of the voice that retain the features that the researchers are interested in but remove the sounds and the words spoken. The goal was to make it difficult to use the data to glean information that identified the speaker and associated them with private information that their utterances might include. It wasn’t long before a researcher at the Massachusetts Institute of Technology in Cambridge told Bensoussan that they’d developed an algorithm that could convert the spectrogram back into speech. The voice was robotic, so it didn’t identify the speakers, but everything that they had said was restored. In response, the project removed access to open-ended speech and included only people reading pre-approved text.
There’s also concern that AI could be applied to speech to uncover behavioural information that a person might not want to disclose14. In 2024, researchers showed that they could apply AI to voice recordings to differentiate between smokers and non-smokers, with an accuracy of 71% for women and 65% for men15. Using such systems to verify what people tell their physicians could undermine physician–patient trust, which might interfere with treatment.
The field of voice biomarkers is advancing rapidly, Bensoussan says. There are already companies that market systems, including Canary Speech, a start-up in Provo, Utah, selling what it calls clinical-decision support systems to alert physicians to signs of cognitive problems. Of course, for anything to be marketed as a diagnostic tool, it would have to receive regulatory approval from the US Food and Drug Administration (FDA) or a similar body. “Nobody has FDA approval right now,” Bensoussan says. How the FDA operates might change as the Trump administration cuts staff and changes the agency’s goals.
More such tools are in the offing, she says. “They’re gonna trickle in, I think, over the next two, three years. And then probably in three to five, we’ll have a lot more presence in the clinic.” She pauses, then adds, “If it works.”