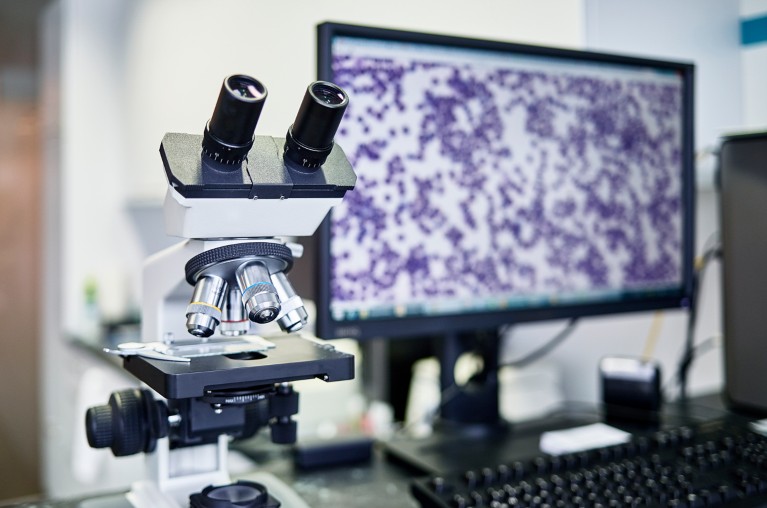
The increasing digitization of microscope slides is making pathology more amenable to advances in AI.Credit: Getty
If you’ve ever had a biopsy, you — or at least, your excised tissues — have been seen by a pathologist. “Pathology is the cornerstone of diagnosis, especially when it comes to cancer,” says Bo Wang, a computer scientist at the University of Toronto in Canada.
But pathologists are increasingly under strain. Globally, demand is outstripping supply, and many countries are facing shortages. At the same time, pathologists’ jobs have become more demanding. They now involve not only more and more conventional tasks, such as sectioning and staining tissues, then viewing them under a microscope, but also tests that require extra tools and expertise, such as assays for genes and other molecular markers. For Wang and others, one solution to this growing problem could lie in artificial intelligence (AI).
AI tools can help pathologists in several ways: highlighting suspicious regions in the tissue, standardizing diagnoses and uncovering patterns invisible to the human eye, for instance. “They hold the potential to improve diagnostic accuracy, reproducibility and also efficiency,” Wang says, “while also enabling new research directions for mining large-scale pathological and molecular data.”
ChatGPT for science: how to talk to your data
Over the past few decades, slides have increasingly been digitized, enabling pathologists to study samples on-screen rather than under a microscope — although many still prefer the microscope. The resulting images, which can encompass whole slides, have proved invaluable to computer scientists and biomedical engineers, who have used them to develop AI-based assistants. Moreover, the success of AI chatbots such as ChatGPT and DeepSeek has inspired researchers to apply similar techniques to pathology. “This is a very dynamic research area, with lots of new research coming up every day,” Wang says. “It’s very exciting.”
Scientists have designed AI models to perform tasks such as classifying illnesses, predicting treatment outcomes and identifying biological markers of disease. Some have even produced chatbots that can assist physicians and researchers seeking to decipher the data hidden in stained slices of tissue. Such models “can essentially mimic the entire pathology process”, from analysing slides and ordering tests to writing reports, says Faisal Mahmood, a computer scientist at Harvard Medical School in Boston, Massachusetts. “All of that is possible with technology today,” he says.
But some researchers are wary. They say that AI models have not yet been validated sufficiently — and that the opaque nature of some models poses challenges to deploying them in the clinic. “At the end of the day, when these tools go into the hospital, to the bedside of the patient, they need to provide reliable, accurate and robust results,” says Hamid Tizhoosh, a computer scientist at Mayo Clinic in Rochester, Minnesota. “We are still waiting for those.”
Building foundations
The earliest AI tools for pathology were designed to perform clearly defined tasks, such as detecting cancer in breast-tissue biopsy samples. But the advent of ‘foundation’ models — which can adapt to a broad range of applications that they haven’t been specifically trained for — has provided an alternative approach.
Among the best-known foundation models are the large language models that drive generative-AI tools such as ChatGPT. However, ChatGPT was trained on much of the text on the Internet, and pathologists have no comparably vast resource with which to train their software. For Mahmood, a potential solution to this problem came in 2023, when researchers at tech giant Meta released DINOv2, a foundation model designed to perform visual tasks, such as image classification1. The study describing DINOv2 provided an important insight, Mahmood says — namely, that the diversity of a training data set was more important than its size.
By applying this principle, Mahmood and his team launched, in March 2024, what they describe as a general-purpose model for pathology, named UNI2. They gathered a data set of more than 100 million images from 100,000 slides representing both diseased and healthy organs and tissues. The researchers then used the data set to train a self-supervised learning algorithm — a machine-learning model that teaches itself to detect patterns in large data sets. The team reported that UNI could outperform existing state-of-the-art computational-pathology models on dozens of classification tasks, including detecting cancer metastases and identifying various tumour subtypes in the breast and brain. The current version, UNI 2, has an expanded training data set, which includes more than 200 million images and 350,000 slides (see go.nature.com/3h5qkwb).
A second foundation model designed by the team used the same philosophy regarding diverse data sets, but also included images from pathology slides and text obtained from PubMed and other medical databases3. (Such models are called multimodal.)
Artificial intelligence in digital pathology — time for a reality check
Like UNI, the model — called CONCH (for Contrastive Learning from Captions for Histopathology) — could perform classification tasks, such as cancer subtyping, better than could other models, the researchers found. For example, it could distinguish between subtypes of cancer that contain mutations in the BRCA genes with more than 90% accuracy, whereas other models performed, for the most part, no better than would be expected by chance. It could also classify and caption images, retrieving text in response to image queries and vice versa, to produce graphics of the patterns seen in specific cancers. However, it was not as accurate in these tasks as it was for classification. In head-to-head evaluations, CONCH consistently outperformed baseline approaches even in cases where very few data points were available for downstream model training.
UNI and CONCH are publicly available on the model-sharing platform Hugging Face (see go.nature.com/44g24w2). Researchers have used them for a variety of applications, including grading and subtyping neural tumours called neuroblastomas, predicting treatment outcomes and identifying gene-expression biomarkers associated with specific diseases. With more than 1.5 million downloads and hundreds of citations, the models have “been used in ways that I never thought people would be using them for”, Mahmood says. “I had no idea that so many people were interested in computational pathology.”
Other groups have developed their own foundation models for pathology. Microsoft’s GigaPath4, for instance, is trained on more than 170,000 slides obtained from 28 US cancer centres to do tasks such as cancer subtyping. mSTAR (for Multimodal Self-taught Pretraining), designed by computer scientist Hao Chen at the Hong Kong University of Science and Technology and his team, folds together gene-expression profiles, images and text5. Also available on Hugging Face (see go.nature.com/3ylmauf), mSTAR was designed to detect metastases, to subtype cancers and to perform other tasks.
Now, Mahmood and Chen’s teams have built ‘copilots’ based on their models. In June 2024, Mahmood’s team released PathChat — a generalist AI assistant that combined UNI with a large language model6. The resulting model was then fine-tuned with almost one million questions and answers using information derived from articles on PubMed, case reports and other sources. Pathologists can use it to have ‘conversations’ about uploaded images and generate reports, among other things. Licensed to Modella AI, a biomedical firm in Boston, Massachusetts, the chatbot received breakthrough-device designation from the US Food and Drug Administration earlier this year.
Similarly, Chen’s team has developed SmartPath, a chatbot that Chen says is being tested in hospitals in China. Pathologists are going head to head with the tool in assessments of breast, lung and colon cancers.
Beyond classification tasks, both PathChat and SmartPath have been endowed with agent-like capabilities — the ability to plan, make decisions and act autonomously. According to Mahmood, this enables PathChat to streamline a pathologist’s workflow — for example, by highlighting cases that are likely to be positive for a given disease, ordering further tests to support the diagnostic process and writing pathology reports.
Foundation models, says Jakob Kather, an oncologist at the Technical University of Dresden in Germany, represent “a really transformative technological advancement” in pathology — although they are yet to be approved by regulatory authorities. “I think it’ll take about two or three years until these tools are widely available, clinical proven products,” he says.
An AI revolution?
Not everyone is convinced that foundation models will bring about groundbreaking changes in medicine — at least, not in the short term.
One key concern is accuracy. Specifically, how to quantify it, says Anant Madabhushi, a biomedical engineer at Emory University in Atlanta, Georgia. Owing to a relative lack of data, most pathology-AI studies use a cross-validation approach, in which one chunk of a data set is reserved for training and another for testing. This can lead to issues such as overfitting, meaning that an algorithm performs well on data similar to the information that the model has encountered before but does poorly on disparate data.
“The problem with cross-validation is that it tends to provide fairly optimistic results,” Madabhushi explains. “The cleanest and best way to validate these models is through external, independent validation, where the external test set is separate and distinct from the training set and ideally from a separate institution.”
Why tumour geography matters — and how to map it
Furthermore, models also might not perform as well in the field as their developers suggest they do. In a study published in February7, Tizhoosh and his colleagues put a handful of pathology foundation models to the test, including UNI and GigaPath. Using a zero-shot approach, in which a model is tested on a data set it hasn’t yet encountered (in this case, data from the Cancer Genome Atlas, which contains around 11,000 slides from more than 9,000 individuals), the team found that the assessed models were, on average, less accurate at identifying cancers than a coin toss would be — although some models did perform better for specific organs, such as the kidneys.