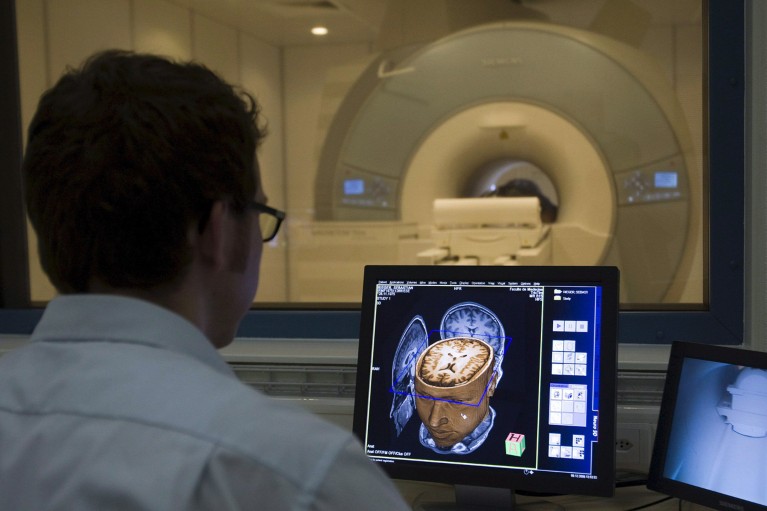
Magnetic resonance imaging scanning at the Brain and Behaviour Laboratory at the University of Geneva, Switzerland.Credit: Salvatore Di Nolfi/EPA/Shutterstock
‘Experimental design’: these words signal a section of a research paper that many readers might be inclined to scan fleetingly, before moving on to the actual findings. But a study in Nature this week should make all researchers — both readers and writers of papers — consider dwelling a little more on the methods part of the scientific process.
Read the paper: Study design features increase replicability in brain-wide association studies
The study, led by Simon Vandekar, a biostatistician at Vanderbilt University Medical Center in Nashville, Tennessee, is on how to make brain-wide association studies (BWAS) more robust (K. Kang et al. Nature https://doi.org/10.1038/s41586-024-08260-9; 2024). The core idea of BWAS is to study collections of brain images using statistical tools and machine-learning algorithms. This is to predict what specific brain features or patterns of activity are associated with traits or behaviours, for example an ability to reason abstractly or a tendency to experience particular negative emotions.
But BWAS have a perennial, and well-known, problem of low replicability: two studies on the same topic can come to different conclusions. Much of the problem is that some BWAS studies need huge sample numbers to reflect effects accurately. Small sample sizes can exaggerate the relationship of a certain brain feature to a behaviour or trait. In the similar field of genome-wide association studies — which seek to relate differences in DNA with traits in health or disease — the problem of unreliability is being overcome by gathering data sets with tens of thousands of samples from participants. However, in the case of the brain, this is much more difficult, especially for researchers outside Europe and the United States. One hour of scanning in a molecular resonance imaging (MRI) machine costs about US$1,000. The US National Institutes of Health distributes around $2 billion for neuroimaging research each year, but few other countries have this level of resource.
Vandekar and his colleagues suggest that concentrating on quality, rather than quantity, could be one answer. They analysed more than 100,000 MRI scans from healthy adults and healthy children, as well as scans from children with mental-health conditions.
Design tips for reproducible studies linking the brain to behaviour
Their aim was to explore how factors such as age, sex, cognitive function and mental health are associated with brain structure and function across diverse study designs. For example, one study explored how brain volume changes with age. Vandekar and his co-authors found that, compared with one-off scans of multiple people — cross-sectional studies — repeated MRI scans of the same people over time yielded more-robust results (see R. J. Chauvin and N. U. F. Dosenbach Nature https://doi.org/10.1038/d41586-024-03650-5; 2024).
Such longitudinal studies have long proved their worth in areas of science such as identifying biomarkers for chronic or degenerative diseases (Y. Guo et al. Nature Aging 4, 247–260; 2024). Although they don’t work for some types of question for which cross-sectional studies are needed, longitudinal studies are good at ruling out irrelevant factors that looked as if they might be implicated during small cross-sectional studies.
There are caveats, however: researchers conducting longitudinal studies must, for instance, take care to leave long enough gaps between measurements in any one individual if they are to capture meaningful and statistically significant differences over time. Vandekar and his colleagues also emphasize that researchers must account both for changes that happen in individuals over time and for differences between individuals.
All research needs to be planned. For BWAS, selecting participants in such a way as to achieve robust results and using the right statistical models can improve the trustworthiness of findings without an automatic need for massive sample sizes. The benefits of statistical rigour, in turn, highlight the need for more collaboration between statisticians and neuroscientists, as they use more-sophisticated data-handling methods in their research. These findings will be valuable to the neuroscience community, and deserve wider attention.
Many fields of science are plunging into data-driven discovery, increasingly assisted by the pattern-seeking abilities of artificial-intelligence algorithms. As they do so, questions of correlation and causation, and of ensuring that findings are statistically significant and reproducible, are becoming ever more pertinent. That means researchers must not skate over experimental design, whether they are reading a paper or writing one.
Greater attention to research methods, and to how a study obtains the signal of its effect, is the way to make sure that results stand the test of time.