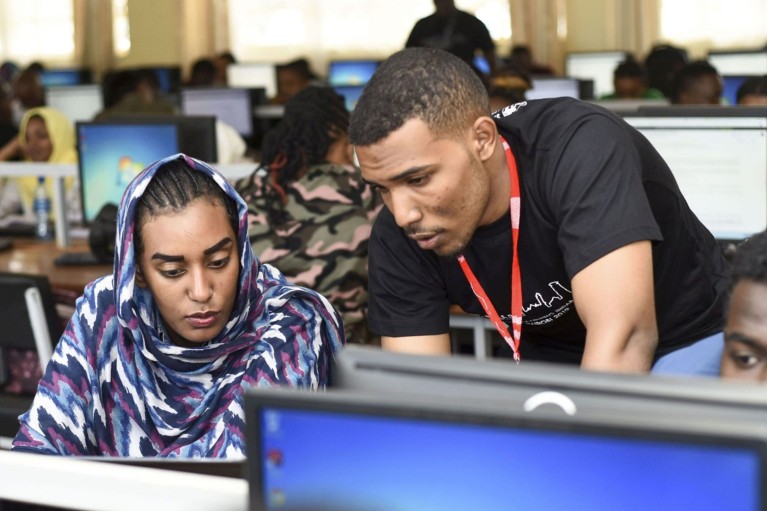
Researchers at a workshop in Nairobi put on by Deep Learning Indaba, a non-profit organization with a mission to ensure that Africans are active developers of AI technologies.Credit: Deep Learning Indaba
The rapid growth of artificial intelligence (AI) offers immense potential for scientific advancements, but it also raises ethical concerns. AI systems can analyse vast data sets, detect patterns, optimize resource use and generate hypotheses. And they have the potential to help address global challenges including climate change, food security and diseases. However, the use of AI also raises questions related to fairness, bias and discrimination, transparency, accountability and privacy. Image-generating AI programs can perpetuate and amplify biases, such as associating the word ‘Africa’ with poverty, or ‘poor’ with dark skin tones. And some technology giants fail to disclose important information about their systems, hindering users’ efforts towards accountability.
Four researchers from different countries give their perspectives on the significant promise and pitfalls of AI used in scientific research. They discuss the need for data sets that accurately represent populations in their entirety, and the importance of understanding the limitations of AI tools. Experts from Africa caution that AI systems should benefit all, and not further increase inequities between richer and poorer countries.
ROSS KING: Harness AI for good, keep ethical standards high
Computer scientist at the University of Cambridge, UK, and at Chalmers Institute of Technology in Gothenburg, Sweden.
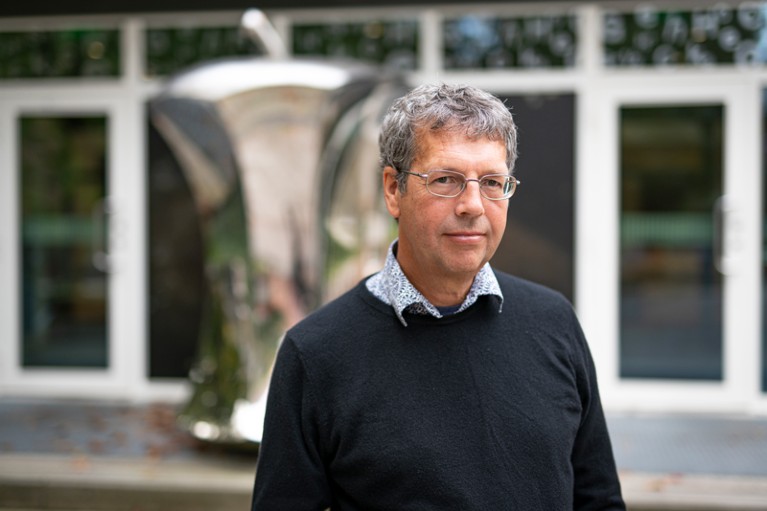
Ross King used a robot scientist to discover that a common ingredient in toothpaste is a potent anti-malaria drug.Credit: Chalmers University of Technology
I’ve been in the AI field since 1983, when I worked on a computing project to model microbial growth for my honour’s degree thesis project. I’m so inspired by AI’s potential that I’ve helped to organize the Turing AI Scientist Grand Challenge, an initiative to develop AI systems capable of producing Nobel prize-worthy research results by 2050. Science has been hugely transformative in human history: billions of people have much better standards of living than previous kings of England had, with better food and health care, global travel and digital communication. But there are still huge problems, such as climate change, pandemics and extreme poverty.
I’ve spent my career in AI trying to make science more efficient. In 2009, my colleagues and I built a robot scientist called Adam, the first to automate scientific research in yeast genomics. Introduced in 2015, Eve — Adam’s better-designed successor — automates early-stage drug design with a particular focus on neglected tropical diseases. We demonstrated that AI reduced development costs, and the approach has now been widely copied across the pharmaceutical industry. Eve discovered that the compound triclosan, a common ingredient in toothpaste, was a potent anti-malaria drug (E. Bilsland et al. Sci. Rep. 8, 1038; 2018).
Why AI’s diversity crisis matters, and how to tackle it
Only in the past few years has anyone really begun to raise concerns about the ethical consequences of AI. Already, it is a bit too late. If the Turing Challenge succeeds, we would have agents that could transform science but that might also have the potential do bad things. That prompted a group of us at a Challenge workshop in 2023 to prepare the Stockholm Declaration on AI for Science. Along with other signatories, we commit to using AI in science for good, and affirm that it should help to meet the great challenges that the world faces, such as climate change and food insecurity. We also recognize the need for rigorous oversight, accountability and safeguards against potential misuse.
We hope that the declaration raises awareness about the pitfalls of using AI. For example, it is important to avoid bias and discrimination. If the training data are not representative of a whole population, then the system won’t generalize properly.
In a similar way, we need to be careful about some of the conclusions drawn by AI systems. Historically, more Black people in the United States have been incarcerated in prisons than have white people, and that has a lot to do with the US history of systemic racism. The reason for this discrepancy isn’t to do with biology — that’s just obvious — but an AI system trained on a data set of incarceration statistics might conclude, incorrectly, that it is. Be very careful that you don’t believe everything that a large language model says, and check the outputs. You are still responsible for your science. You can’t just say, “AI told me to do it.”
I don’t think there’s anything ethically worrying about using AI to process your data, generate hypotheses or suggest an experiment. It is just a tool. Ultimately, let your conscience be your guide.
SURESH VENKATASUBRAMANIAN: Understand the limitations of AI tools
Computer scientist at Brown University in Providence, Rhode Island.
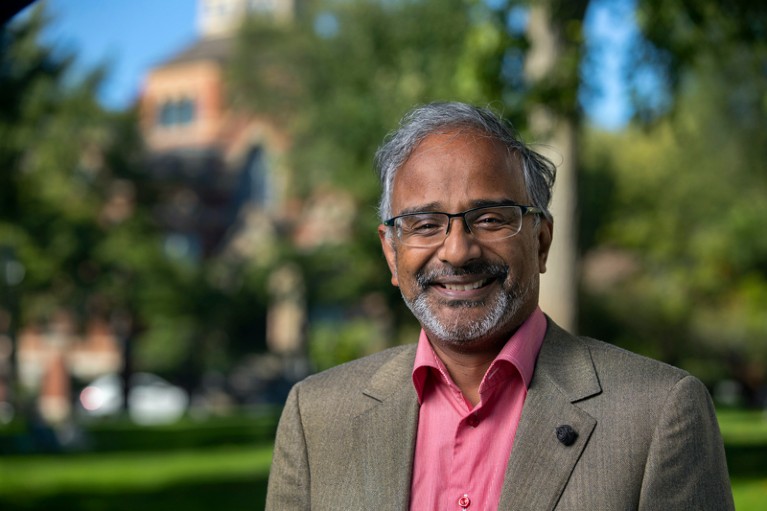
Suresh Venkatasubramanian helped to co-author the first US blueprint for an AI Bill of Rights.Credit: Nick Dentamaro/Brown University
On a sabbatical in 2013, I started thinking about AI in a big-picture way, asking myself, “What happens if we are using machine learning everywhere in the world?” and “How will we know these systems are doing what they are supposed to be doing?” Now, I focus on the effects of automated decision-making systems in society and, in particular, I investigate algorithmic fairness.
Algorithmic unfairness describes what happens when algorithms that are used in decision-making lead to decisions based on characteristics that we don’t think should play a part. For example, an algorithm that assists in recruitment decisions for a tech company might favour men over women.
Not all ‘open source’ AI models are actually open: here’s a ranking
In 2021, I was asked to serve as the assistant director for science and justice in the White House Office of Science and Technology Policy and to help co-author the first US blueprint for an AI Bill of Rights. The document outlines five core principles to protect people, including data privacy and avoidance of algorithmic discrimination. It is relevant for scientists, especially when their use of AI in research affects civil rights, perhaps by influencing opportunities for advancement or access to services. As a biomedical researcher, for example, you might be making medical devices or designing treatment plans that affect people’s lives and their ability to function in society.
The AI Bill of Rights will provide more concrete advice to AI practitioners. We need better guidance in the capabilities and limitations of AI tools. All tools have limits. You don’t use a screwdriver to hammer a nail, unless you’re desperate.
Much of the public discussion around AI is at a high level, where it’s just not helpful. A researcher needs to be very specific and people-centred or they won’t really get a deep understanding of the broader societal impacts of the tools they’re creating. For example, developing an intricate and fair assessment tool to predict who might be least likely to show up for their court dates, and then targeting them, could be less effective than simply sending reminder messages to everyone about their trial dates.
At Brown University, where I direct the Center for Technological Responsibility, one thing we think about is how to educate a wide swathe of researchers about what AI can and cannot do.
Can we use ChatGPT to generate title ideas for an article? Sure. Can we use a chatbot to get accurate answers to questions? Not right now, but stay tuned. Can we use AI to make critical life-altering decisions? Almost certainly not, and at least not without significant protections and safeguards. Right now, we are in the hype cycle, in which no one’s talking about the limits of these tools, but it’s important to convey a more balanced sense of what these tools are good for, and what they’re not good for.
NYALLENG MOOROSI: Effective, ethical AI requires representative data
Computer scientist at the Distributed AI Research Institute in Hlotse, Lesotho.
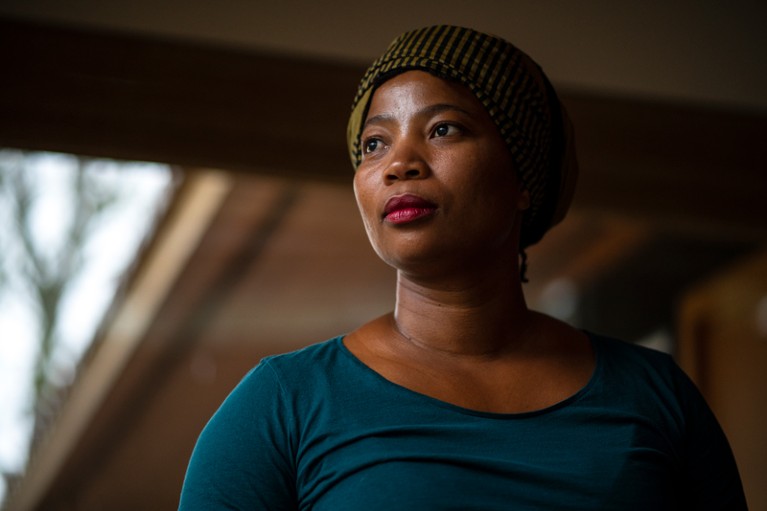
Nyalleng Moorosi co-founded Deep Learning Indaba so that Africans could learn to become ‘active shapers and owners’ of advances in AI.Credit: Nik West
In 2016, I was working on a data-science project for the South African government’s Council for Scientific and Industrial Research, using social-media data to understand political trends and sentiments in that year’s local elections. It was clear that most of the conversations were being led by urban, well-to-do voters. We didn’t have the voice of the rural populations, and there was little representation from older or low-income populations. We also realized that we could access information that people wouldn’t necessarily wish to volunteer, such as their locations or details about family and friends. All these issues of equity and privacy really came to light. This developed my passion for data representation: that is, who is included in the data sets and how they are portrayed.
Data representation is really important in Africa. In the large language models that power AI chatbots, there are very few resources for most African languages, so the models perform badly on tasks such as language identification and translation. A case in point is a paper published at the North American Chapter of the Association for Computational Linguistics 2024 conference. The paper shows that, when used to identify 517 African languages in a specific data set, ChatGPT averaged an accuracy of only 5% (W.-R. Chen et al. Preprint at arXiv https://doi.org/ndmd; 2024). But it was able to identify English 77% of the time. AI is all about historical data for training, and these AI systems don’t work for African countries, because they don’t have data from those countries. Developers effectively haven’t ‘seen’ us.
AI image generators often give racist and sexist results: can they be fixed?
When African AI developers get these poorly performing systems, we rush into our communities and we build these data sets. We interact with these systems, and we correct them, and the systems learn, learn, learn. The best-case scenario is that we get the systems to a place where they do work for us, but because we don’t own any of the companies behind them, we have done all of that work for free.
Instead, we should be focusing our resources on local researchers and developers so that we can develop our own systems. Then we’ll be able to use our own metrics of correctness and incorporate meaningful data. Masakhane, one of the largest language-model-building communities in Africa, has now constructed several benchmark data sets on which lots of other local language tools have been built.
Another problem with AI is that it can produce outcomes that societies cannot tolerate, such as when the Google Photos app labelled two black people as gorillas. Now, Google has created systems that will never do that, by removing the label ‘gorilla’ from their data sets. Of course, this might not seem like an optimal solution to us in the research community, but it goes to show how intolerable that mistake was in the United States. Developers and companies put themselves, their culture and their politics in there. That’s why it is important to build AI systems locally, because we know what we are sensitive to and what makes sense to our communities.
My AI developer colleagues and I felt that we, as Africans, needed to learn enough to develop these technologies for ourselves. So, in 2017, we formed the non-profit organization Deep Learning Indaba — indaba is the Zulu word for gathering — to strengthen machine learning and AI in Africa. At first, we just ran a summer school, to which people would come to learn about the fundamentals of machine learning. Now the organization does much more. Our goal is that Africans will be not only observers and receivers of advances in AI, but also active shapers and owners of those advances. We know our problems, and we are great at solving them.
SEYDINA NDIAYE: Prevent AI-driven colonization in Africa
Programme director and lecturer at the Cheikh Hamidou Kane Digital University in Dakar, Senegal.
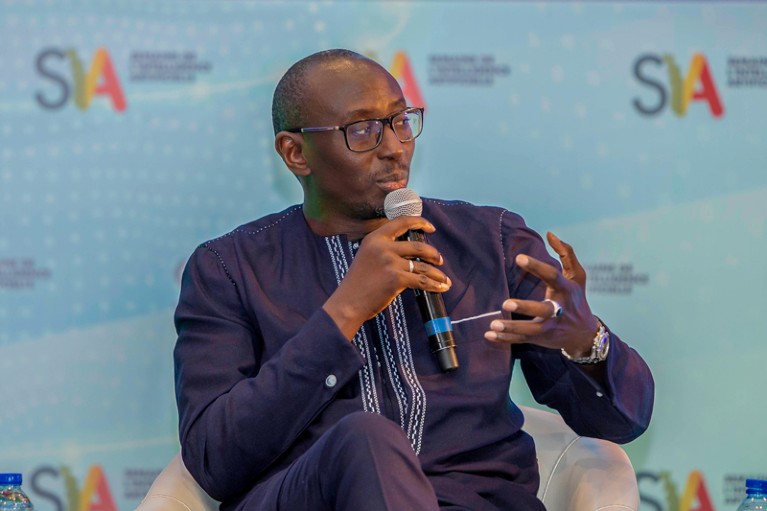
Seydina Ndiaye is concerned that Africa is seen as full of AI resources that could be exploited.Credit: NGH CORP
For my PhD, I worked at the French National Institute of Agronomic Research in Toulouse on the use of AI for optimizing the farming of winter wheat. The positive potential of AI in Africa is enormous, particularly if analysed through the lens of the United Nations’ 17 Sustainable Development Goals, which call for simultaneous efforts to address poverty, hunger, disease and environmental degradation. The advances of AI in areas such as agriculture, health and education mean that it is already possible to apply this technology to solve many of Africa’s common problems. AI also provides a real opportunity to develop and strengthen African cultural identity in all its diversity. People in the African AI community are already seeing enthusiasm to produce African-specific content that can be shared with the rest of the world. And we’re seeing African-led AI systems for African languages.
After my PhD, I corresponded with former undergraduate classmates and professors at the University of Senegal to promote the idea that we needed to set up local IT companies to ensure our sovereignty in this field — and not just be consumers of imported products. We created several IT education and software-development companies, including SeySoo, which provides IT services and training in Senegal, Gabon, Burkina Faso and France.
At the beginning of my career, I saw AI only from a positive point of view. But in the past 15 years, the AI boom has been guided more by economic gain than by solving the problems facing humanity, and I’ve started to question that perspective.
Rules to keep AI in check: nations carve different paths for tech regulation
I am concerned that Africa is being managed, against its will, as the least rewarded link in the global chain of the AI economy by the big powers in the field. They see Africa as a source of health data that are difficult to obtain elsewhere, or of low-paid workers for sorting and labelling data. I am concerned about it being colonization all over again. When we talk about colonization in Africa, we think of gross exploitation of natural and human resources, but the most negative impacts of colonization are undoubtedly the loss of the cultural identity and of initiative, particularly in technological innovation.
The race for AI supremacy hinges on three main pillars: computing power, talent and data. Thanks to international competition and increasing global demand, these resources are becoming scarcer. Countries and major corporations from the global north tend to view the global south as untapped territory rich in talent and data. What is in danger of happening — and this has already begun — is that the African continent will be stripped of its most experienced human resources through emigration, and Africans who are employed as data workers will be exploited with poor working conditions, low wages and little job security.
In international research projects, we often see that African partners are used to provide data to build models, or to facilitate frameworks for large-scale experimentation. This reinforces colonialist tendencies. But if, instead, African researchers could be recognized as making a significant contribution to the AI scientific process, we could end up with innovative solutions that would be the fruit of different world perspectives. InstaDeep, a start-up with offices worldwide, created and run by Africans, has made considerable advances in AI-powered drug discovery, design and development and has subsequently been acquired by biotech firm BioNTech.
For researchers using data collected in African countries, it is important to respect confidentiality, especially regarding personal details, such as health measures. It is also important to ensure that the Africans who contributed data for AI models get to benefit from those systems.
If we want responsible AI for all, and by all, it is crucial to make sure that the African continent is not left behind. This is mainly the responsibility of African policymakers, who need to make AI a priority and provide the necessary resources for African researchers and entrepreneurs to innovate. But the international research community can also help, by including African expertise and experience in projects, and by carefully considering how African data are used. Global partners can help African researchers to be architects of amazing AI solutions for Africa and the world.